Five Mistakes to Avoid When Starting an AI Project
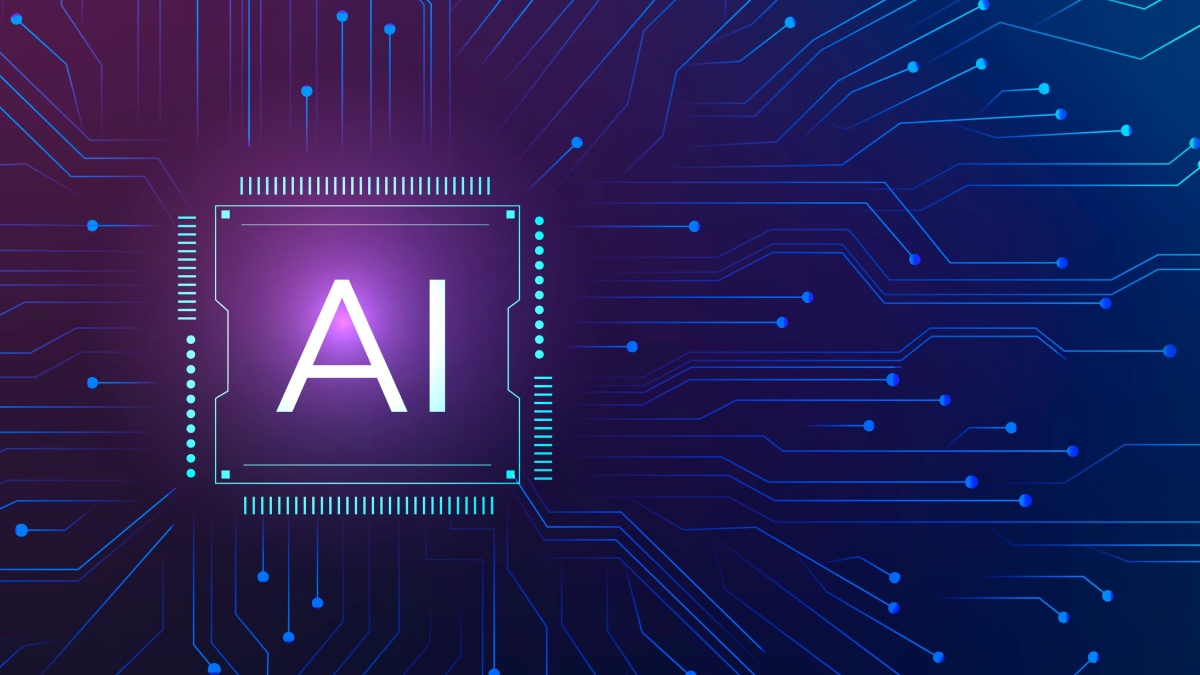
WekaIO knows that there are some things that can make a huge difference in our customers’ success with their AI/ML projects in production. We know that storage is challenged with keeping up with the requirements of each stage in the AI pipeline. In a recent conversation with Julia Palmer from Gartner we learned about five mistakes that companies need to avoid when starting their AI/ML projects.
1. Use Shared Storage
We know that direct-attached storage does not scale. Use shared storage solutions that consolidate data platforms to avoid data silos and eliminate the need for you to manually move data between pipeline stages. This approach dramatically improves your storage efficiency and data management.
2. Modernize Your Storage Network
Obviously, you want to use the latest flash technologies, but also consider leveraging the latest protocols, such as RDMA NVMe-oF for performance efficiency. You don’t want the fabric to become a bottleneck for the infrastructure.
3. Minimize I/O Bottlenecks
Bottlenecks can be moving targets that you can spend too much time chasing because oftentimes AI practitioners fix a bottleneck on one side of the solution, only to find that it moves to the other side. If you design your AI/ML infrastructure as one solid, end-to-end solution from the beginning you won’t need to spend precious time figuring out how to fix storage deployment bottlenecks mid-stream, and you can leverage the full value of your investments, especially the specialized hardware. You can keep those data-hungry GPUs fed.
4. Seek Consistent Linear Performance
As you add capacity you need to add performance, so it’s important to run extended POCs that test the performance at scale across your mixed workloads. Admittedly, on Day One your performance may be great, but as you add more storage, such as NVMe drives, you can quickly saturate the controller, and your CPUs will not be able to support the storage media. From Day One you need to think about how you’re going to grow performance at scale.
5. Evaluate All Options
Let’s face it: AI/ML workloads ingest data constantly, so you need to think about what your solution will look like as it scales to 1PB, 10PB, 20PB, and more. Look at your storage infrastructure holistically by evaluating all essential elements: portability, deployment options, interoperability requirements, scale, and cost. Ignoring one in the early stages of a project can cause frustration and costs to mount as the project progresses. Will it work for bare metal deployments? Will it work for Kubernetes? Ask the right questions up front.
Conclusion
Bottom line: proper preparation at the early stages of an AI project can make the difference between early success and a drawn-out, frustrating effort. Do yourself a favor and map out a plan to avoid common pitfalls.
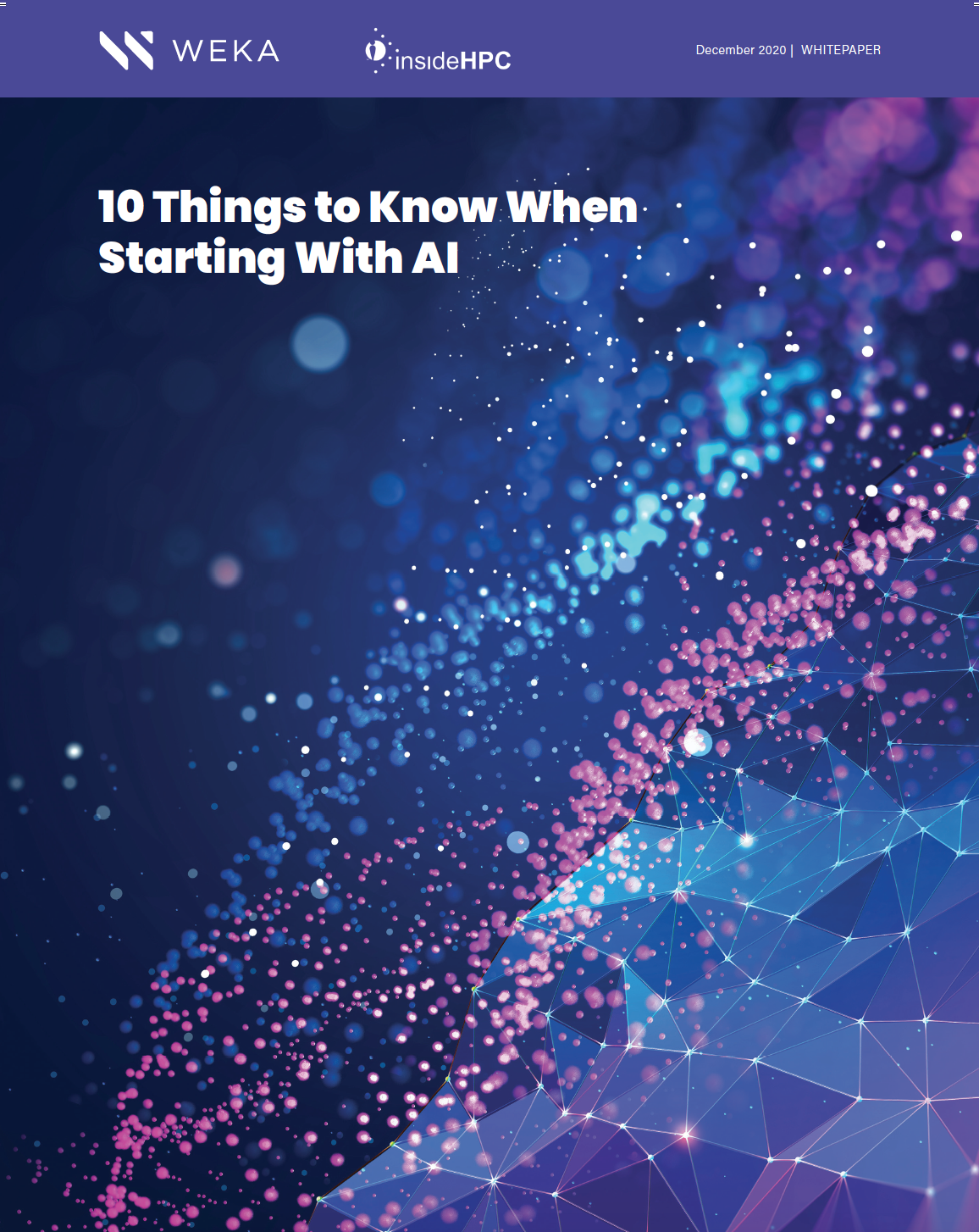
10 Things to Know When Starting with AI
How to ensure your AI initiative is set for success
Additional Helpful Resources
Six Mistakes to Avoid While Managing a Storage Solution
IDC Report – Requirements of a Modern Storage Platform
Data Management in the Age of AI
Architectural Considerations for AI Workloads
How to Rethink Storage for AI Workloads