Bigger is Better
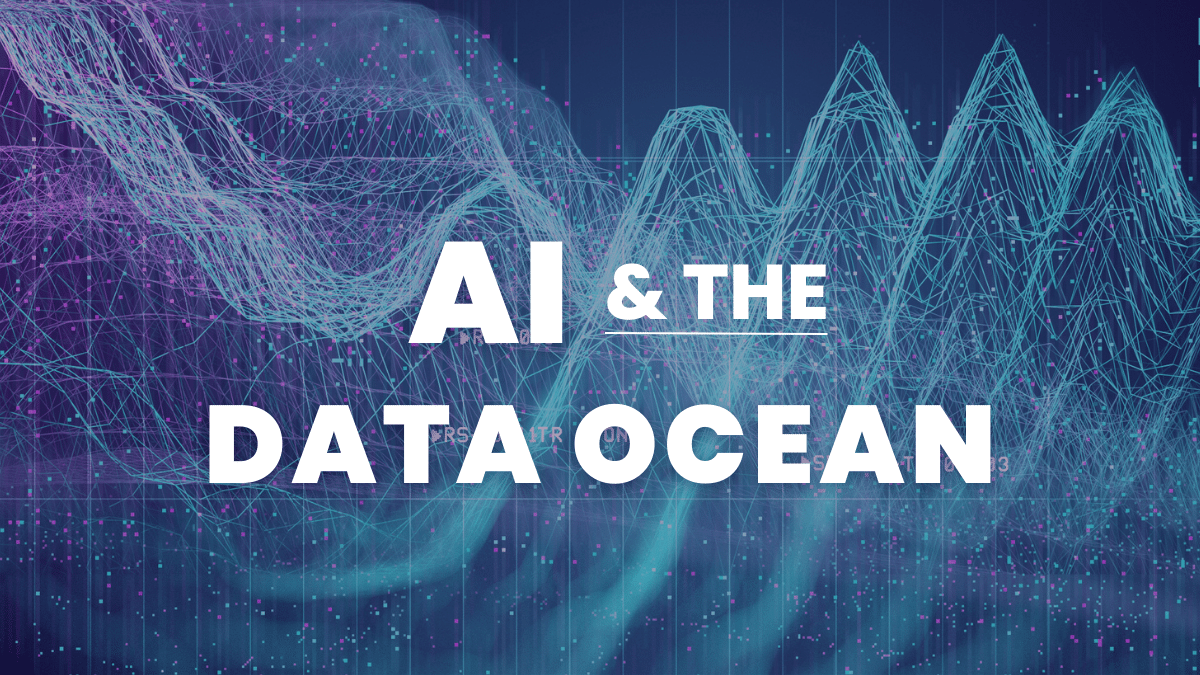
Why AI Needs a Data Ocean
“Leaders must invoke an alchemy of great vision.” – Henry Kissinger
Earlier this year we posted a prediction blog about the imminent rise of data oceans and hinted their necessity will be driven by the global artificial intelligence market. Where the prediction may have been a bit off is when it alluded to the expansion of the data lake to the data ocean. Now, while you can make a mountain out of a molehill, you can’t make an ocean out of a lake. In other words, the use of “expansion of a data lake into a data ocean” was semantically inaccurate, but the concept of outgrowing a data lake and transitioning to a data ocean accurately captures the direction that we’re seeing data-driven enterprises move.Â
Before going any further, let’s talk a little bit about what makes up a data ocean starting with the definition of a data lake. A data lake contains raw, unprocessed data traditionally focused on a specific part of the business, and usually has no real expectation for high performance. By comparison, a data ocean is much more expansive and offers massive scale to retain the same data as a data lake in addition to the small and wide data needed to provide a purview for finer analysis. Small and wide data may be new terms to some of you, so it may be prudent to give a quick summary. Small data is an analytical technique to discover compelling and meaningful insights from smaller, individual data sets, while wide data is all about tying together disparate data sources across a wide range of sources to come up with useful analyses. Wide data may present buying habits or trends of certain customers who purchase particular brands of products, whereas small data could be viewed as the opposite of big data in that it provides a much more narrow analysis of a single focus point, and therefore is much more difficult to extract from big data sets. A great example of small data is what has become known as the “LEGO beat-up sneaker” and it is a very compelling observation of the power organizations are finding with small data insights. A 2021 Gartner, Inc. analysis predicts by 2025, “70% of organizations will shift from focusing on big to small and wide data.” This shift to small and wide data will give customers smaller, more discrete sets of data for greater context and ensure artificial intelligence (AI) has the copious amounts of data it needs to operate optimally.
Businesses, agencies, and organizations have been swimming in massive amounts of data for the past couple of decades, and as the quantities of data continue to grow, finding new ways to use this data has become a challenge. The data lake, a term coined by Jim Dixon, former CTO and founder of Pentaho, was a way to describe a repository where all raw, unprocessed data would be stored in the event it may have value at some point in time. However, the data growth created a “land of data lakes” or silos, and teams quickly found that data lakes without inspection can turn data lakes into “data swamps”.
Taking the plunge
We mentioned at the outset that the global artificial intelligence market would drive the necessity of a data ocean. Let’s dive right in and expand on that assertion. In the early 90s the term “big data” was first used, then by the early 2000s, it was used to explain the growth phenomenon in business analytics solutions driven by the likes of Hadoop and all of the Hadoop-like iterations. Everyone was talking about “big data” and data analytics, but as you undoubtedly have seen, the relevance of Hadoop has dropped significantly as customers want a faster and more discrete analysis of their data and a platform where machine learning and deep learning are possible.
Now, before we go any further, let’s explain the two distinct categories of AI. First, there is Artificial Narrow Intelligence, or ANI, and Artificial General Intelligence, or AGI. ANI is what is generally available in the market today, meaning it is designed to perform a specific task, such as playing chess, recognizing speech via voice assistants such as Alexa, Siri, and Google Home, presenting predictions based on trend data, etc. These ANI solutions or systems are given data and probable outcomes in the training model, creating a very similar result to that of a human responding to a preset script of responses. On the other end of the spectrum, we reach into more of the Artificial General Intelligence (AGI) models like GPT-3 and GPT-4 that have pioneered human-like conversational tools, and more advanced medical expert systems that begin to mimic the human process of learning from the experiences and interactions it has and creates with the data it is presented and the data it produces over time.
Some liken AGI to that of a child learning and processing based on action and reaction. The action may not be prompted by a training model, but the reaction provides the data insights and logic it will apply during the next iteration of this action. While fully realized AGI platforms don’t exist today, AGI is an area of strong future growth. For that growth to happen, it requires not only a deep and expansive repository such as a data ocean but a platform by which that data can be delivered and processed. Two significant components are required to support this type of massive AGI initiative: massive performance, and an exascale capable repository.
The speed and performance of AGI-ready data infrastructure will be crucial – and not just read performance but write performance. Given the earlier example of a child processing data based on action and reaction, there exists a real need not only to read data in, as most ANI solutions do but also to output data at extreme rates to allow the AGI solution to build iteratively on its learning and experiences. Think about the limitations you’d face if your solution could only write at a paltry 5, 10, or 20GB/sec. Not only would that slow down the learning process, but it would also leave your very expensive data infrastructure powered by GPUs essentially idle.
The global artificial intelligence market is expected to show very strong growth over the next 5 – 10 years with a projected market size of nearly $2 trillion by 2030, presumably moving our industry closer and closer to true Artificial General Intelligence (AGI). Staying ahead of the wave by deploying a data ocean as part of your data infrastructure will be key to the success of many organizations that have placed AI/ML as a tier-one initiative on their roadmap.
Conclusion
In the era of big data, making informed decisions requires a robust data management strategy. While data lakes have served as a valuable tool for storing large volumes of data, the concept of the data ocean takes data management and data intelligence to the next level. By combining the breadth of exascale capacities with extremely low latency, high-performance data access, and improved and broad data accessibility, the data ocean provides a more holistic and effective solution for navigating the extensive seas of data. Embracing the data ocean approach enables organizations to unlock the full potential of their data assets and steer confidently toward success in the data-driven world.