What’s Really Driving the Chip Shortage?
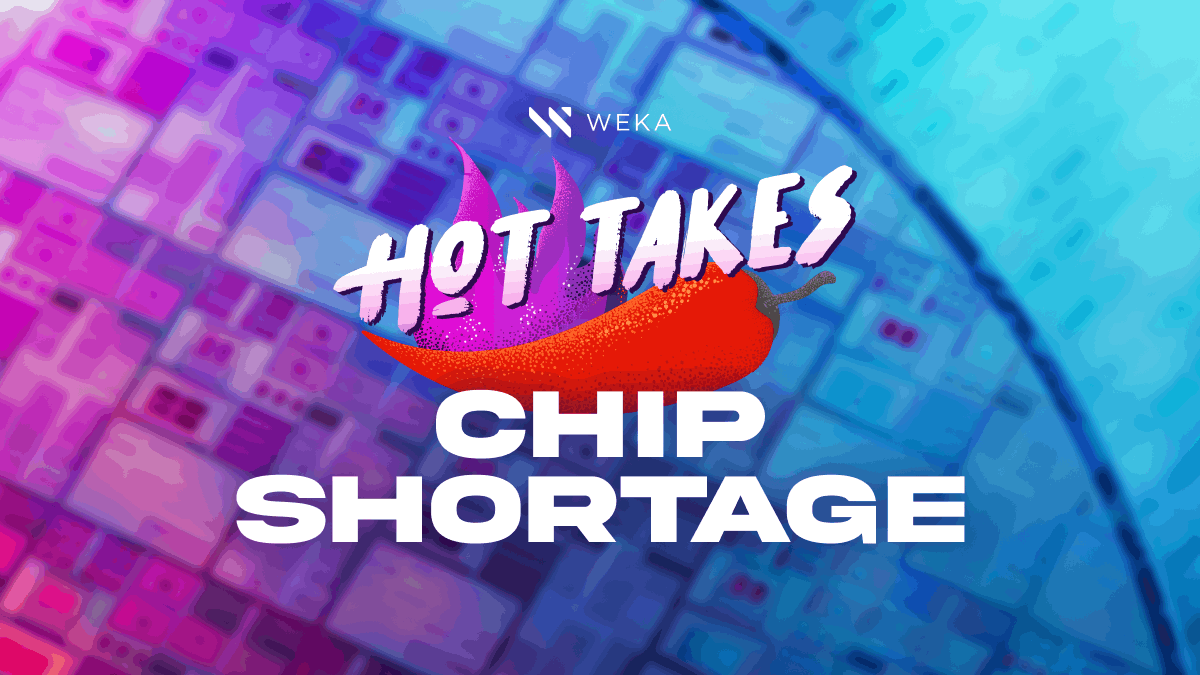
Welcome to WEKA Hot Takes: An op-ed blog series where we share our insights on the latest happenings in the tech industry, the ever-evolving world of AI, and beyond…
Today’s Observation: I’ll take an order of chips with a side of GPUs, please.
It would be nice if companies could simply place such an order with an expectation of near-term delivery, versus the extended delays we all hear about.
What is happening with the market? Why are we still experiencing these chip shortages?
As the generative AI boom continues to gain momentum and splashy headlines by the week, customers and vendors are asking those and many more questions, and it would appear we may have walked right into the middle of a perfect supply chain storm. If you do an internet search asking why we have a chip shortage you will invariably be presented with a variety of articles and opinions. Some attribute it to increased chip demand by the auto industry creating an added layer of pressure on an already burdened chip market. Others are tracing the shortage to surging enterprise demand for GPUs to fuel ambitious AI projects like the generative AI phenom ChatGPT and its increasingly ravenous appetite for more processing power.
Sure, pent-up or increased demand from multiple industries is a contributing factor. But a simple supply and demand imbalance doesn’t tell the whole story. So, what’s really driving the current chip and GPU shortage?
To get a real sense of the issue at its core, we must revist a pandemic-stricken 2020, when business as usual became unusual and supply chains were either backed up or frozen entirely with no definitive end date in sight. Few industries escaped feeling the impact of the pandemic, but it seems the tech space for many reasons was among those hit the hardest. As the world faced unknown circumstances and the phrase “unprecedented times” went mainstream, analysts from all major sectors were trying to locate a directional beacon to provide some sense of optimism and certainty to their respective spaces of influence. By mid-2020 it was projected that the chip shortage would be short-lived, yet in early 2021 analysts extended their projection and said relief was in sight by Q4 and into early Q2 of 2022. The question is, did relief actually arrive in late 2021/early 2022, or did demand increase beyond the relief provided as supply chains regained their pre-pandemic flow? Since we are sitting here in the Summer of 2023 asking why we’re still experiencing a chip shortage, perhaps we have more variables to examine.
As an industry, we’ve been talking about the promise of AI/ML for decades. In fact, artificial intelligence dates to the 1950s when Alan Turing asked the question: why can’t machines think and use reason just as humans do to solve problems? Over the past 70+ years, artificial intelligence experienced a slow climb until the era of Big Data and leveraging analytics for business intelligence in the early to mid-2000s created narrow AI models to aid businesses in decision-making, and AI began to infiltrate the enterprise. But it wasn’t until late 2022 when more advanced general AI emerged in the generative AI space and exploded in popularity – with ChatGPT leading the way and driving AI firmly into the mainstream. Generative AI typically requires much more data and processing power than AI workloads have ever required before, hence the adoption of GPUs for these advanced applications.
In its recent article, The AI Boom Runs on Chips, but It Can’t Get Enough, the Wall Street Journal takes a hard look at the challenges many AI-focused businesses are now facing because of this shortage phenomenon. Co-founder and CEO of Lamini, a startup helping companies build AI models such as chatbots said of GPUs, “It’s like toilet paper during the pandemic.” But there aren’t any signs limiting GPUs to 3 per customer in this case. In fact, shortly after the March AI Open Letter came out signed by many industry luminaries calling for a six-month moratorium on the development of advanced next-gen AI systems, one of the signatories ordered a whopping 10,000 GPUs for work on a generative AI project.
So, back to the chip shortage discussion. Another point to raise is the geopolitical pressures that have limited trade access, thereby forcing increased production at chip-manufacturing facilities already running at capacity. While the United States has invested in rebuilding its position in the semiconductor industry, it only accounts for just 12% of the global semiconductor production capacity, with more than 80% of that capacity from Asia. Not to mention the investment in these new fabs will take quite some time to be fully operational.
So far, we have hit on several contributing factors:
- Increased demand – largely, but not solely – driven by AI applications.
- Pandemic-fueled supply-chain disruptions.
- Limited supplier base, as the complex chips that power AI are manufactured by companies concentrated in just a few countries.
All these variables influence the availability of GPUs to the masses, and when they collide at the same time you get a massive market shortage – hence the reference to the perfect storm. The demand and desire for more GPUs have increased exponentially with the prevalence of next-generation workloads and applications like AI and ML. However, we would be remiss if we failed to mention a bit of research that says GPUs may be left idle for up to 70% of the time. Chalking chip and GPU scarcity up to a clear-cut market shortage assumes that GPUs in the enterprise are being fully utilized. Has the industry been overlooking a little-known but widespread problem? You see, GPU-accelerated data-intensive workloads consume data significantly faster than CPU-based systems, which is why a storage solution designed for legacy workloads will often create a data bottleneck. Studies have shown these bottlenecks may leave GPUs idle for up to 70% of the time. Not only that but from a sustainability perspective, an idle server can still draw as much as 50% of maximum power. The result: wasted energy and wasted GPU capacity.
So, the real questions we should be asking are: Why do all of these AI companies “need” more GPUs? Is it because AI workloads are so data-intensive that they require more GPU power than anticipated, straining the capacity of enterprise tech stacks? Or are most enterprises simply not utilizing their existing GPUs at full efficiency? And is this due to inefficiencies in their data architecture?
When you start peeling back the onion layers, these are interesting questions to consider. If the inefficient utilization of GPUs turns out to be a major source of the chip shortage, then the solution needs to go beyond pulling supply and demand market levers and focus on revamping the data architecture that is serving the GPUs. If you’re still using a legacy data storage solution, chances are you are unable to push the GPU as hard and as fast as you would have liked simply because your storage solution wasn’t designed for the new era, but for workloads dating back several decades ago. Enter the modern data platform, which is designed to eliminate the data siloes and bottlenecks of legacy data management and storage solutions to keep data flowing seamlessly and GPUs fed at optimal efficiency.
TL;DR
The global chip and GPU shortage are complex challenges with multiple contributing factors. Increased demand, supply chain disruptions, and concentration of production have collectively resulted in widespread shortages across industries. This is a perfect time to identify and resolve inefficiencies in your data architecture and ensure your technology investments are delivering optimal value, performance, and efficiencies.
And, if you’re struggling to maximize your existing GPUs and keep them running efficiently, the WEKA® Data Platform can be a key ingredient to help keep them busy and running efficiently, which may help to keep you from having to stand in line for your share of an extremely limited GPU supply.