2023 Global Trends in AI
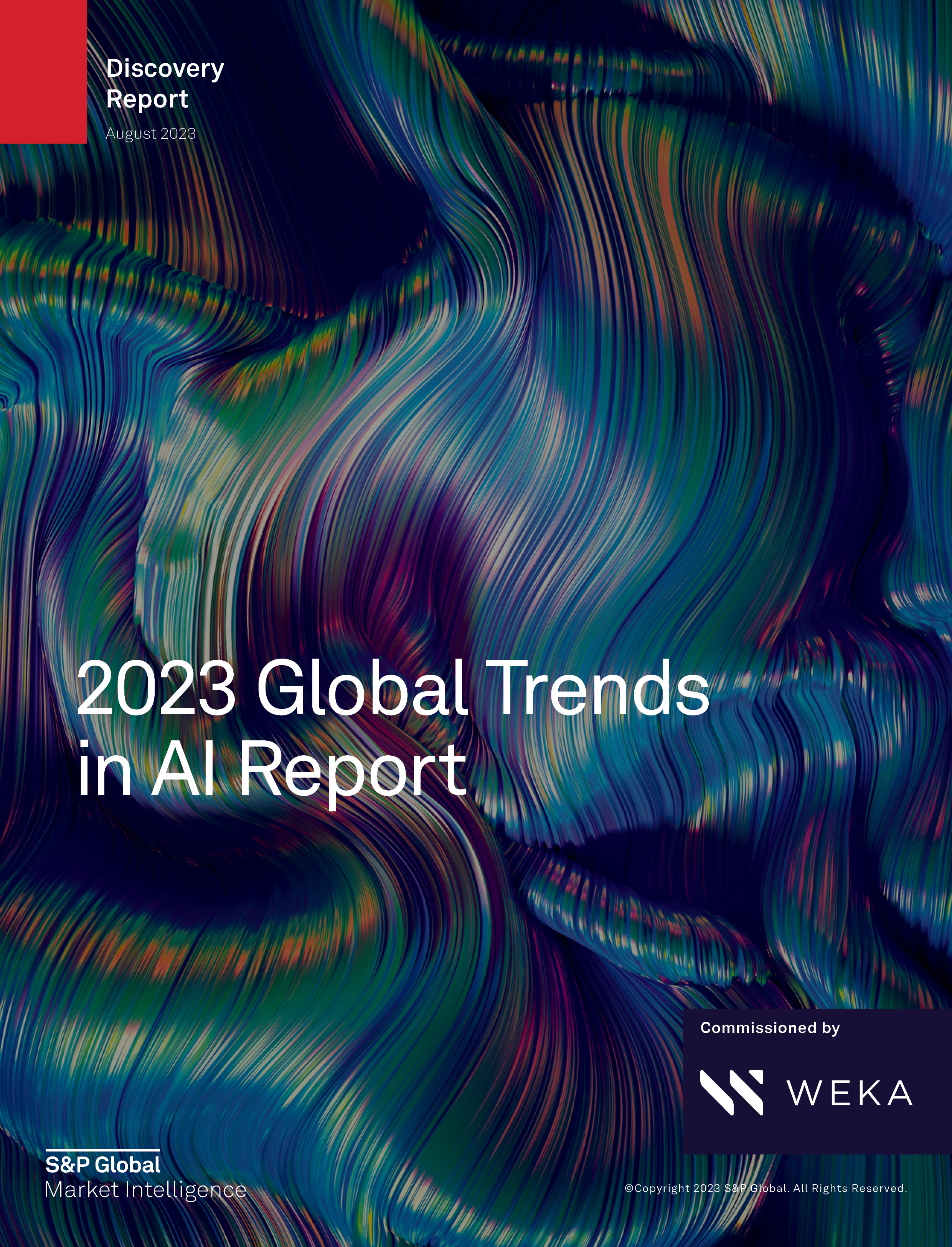
Table of contents
-
Introduction3
- Research overview & key findings3
-
AI is rapidly maturing at enterprise scale, impacting business outcomes5
-
Organizations’ IT infrastructure and data architectures are unfit for the AI revolution7
-
AI and modern infrastructure provide a path to sustainability despite near-term costs9
-
Enterprises with their data/infrastructure houses in order are poised to be future AI leaders12
-
The value derived from AI varies by region, industry and size14
- Aerospace & defense 16
- Automotive 16
- Education 16
- Energy/oil & gas 16
- Financial services 17
- Government 17
- Life sciences 17
- Media & entertainment 17
-
Conclusions 18
-
Methodology 19
-
About the authors 20
Introduction
AI is no longer an emerging technology hinted at in product and strategy roadmaps. This 2023 Global Trends in AI Report finds that the technology is already a catalyst for transformation — 69% of surveyed organizations report having at least one AI project in production, while 28% have reached enterprise scale. These “AI pioneers” have navigated beyond pilot projects and are integrating AI into their workflows and value propositions.
However, with maturity and scale come challenges, and many organizations are acutely feeling the strain that AI models place on their data infrastructure. The increasingly large datasets required to train AI models are composed of diverse data types and have complex computation requirements, pushing the limits of traditional IT and data architectures.
These infrastructure and data challenges are exacerbated by the rapid propulsion of generative AI, which has defined much of the AI market’s evolution in 2023. Around a third of survey respondents leverage generative AI today, showing major adoption in a software space forecast to grow into a $36 billion market by 20281. The significant computing power, storage and networking resources required to train large foundational models have placed organizations in a challenging position, especially as they try to keep pace with generative AI breakthroughs in conjunction with their existing AI deployments.
However, the relationship between AI and IT infrastructure is not purely adverse. Organizations also see machine learning as critical in driving efficiency improvements. A similar pattern emerges with sustainability, with AI both a challenge to and a critical enabler of performance against corporate sustainability goals (CSGs).
While cost savings and efficiencies are undeniably attainable with AI/ML applications, the 2023 Global Trends in AI Report shows that organizations are increasingly looking to AI to help unlock new revenue streams. Leveraging AI/ML to drive top-line growth via new business models, innovative products and differentiated services is causing markets to evolve rapidly.
Research overview & key findings
The Global Trends in AI Report examines the state of AI adoption in 2023 and delivers key data-driven insights on likely developments. It looks at the use cases and value drivers of successful AI projects across industries and the data management and sustainability challenges organizations face as they attempt to scale their AI applications, particularly those that have not invested in modernizing their data architectures and infrastructures.
In support of this study, S&P Global Market Intelligence surveyed over 1,500 AI decision-makers and project leaders worldwide, culminating in seven key findings:
- AI/ML is accelerating across industries and within organizations, but few have reached true enterprise scale. More than two-thirds (69%) of surveyed organizations have at least one AI project in production (“AI pioneers”), whereas 31% of respondents’ AI projects are still in pilot or proof-of-concept stages (“AI explorers”). Additionally, 28% of survey respondents cite reaching enterprise scale with AI projects widely implemented and driving significant business value.
- AI has shifted from a cost-saving measure to a revenue driver, and it is redefining markets. Of 5,400+ responses received from over 1,500 survey respondents, 69% of responses regarding the motivations behind AI/ML projects cite revenue-focused drivers, as opposed to 31% that are cost-focused. Among AI pioneers, 70% of responses correspond to revenue drivers, compared with 66% of responses from AI explorers, suggesting that the focus on AI’s potential to drive revenue increases as AI initiatives mature.
- Data management is the top technical inhibitor to AI/ML. The most frequently cited technological inhibitor to AI/ML deployments is data management (32%), outweighing security challenges (26%) and compute performance (20%). This points to many organizations’ current data architectures being unfit for the AI revolution.
- AI pioneers leverage a hybrid approach and more deployment locations to support the demands of AI/ML workloads. AI/ML workloads can operate in a wide variety of deployment locations, ranging from the public cloud to enterprise datacenters and, increasingly, edge sites.AI pioneers (respondents with AI/ML projects in production environments) leverage more of these locations on average (3.2 deployment locations for training, 2.5 for inference) than AI explorers (2.9 and 2.3 locations, respectively). However, with data-intensive and complex AI applications, the public cloud provides an easier lift to start; the public cloud is the top primary deployment location for training AI/ML models (47%) and inferencing (44%). Additionally, those who use the public cloud to run AI/ML are more likely to use a hybrid approach incorporating more locations for training (4.2 on average) and inference (3.2), as opposed to respondents who do not use the public cloud (2.2 and 1.9 locations, respectively).
- AI/ML’s energy use and carbon footprint are straining corporate sustainability goals, but the cloud presents a path to improvement. Organizations are challenged by AI/ML’s toll on their corporate sustainability goals. More than two-thirds (68%) of respondents indicate they are concerned about the impact of AI/ML on their organization’s energy use and carbon footprint. The cloud provides a path to greater AI sustainability: 74% of total respondents say sustainability is an important or critical motivator for moving workloads to the public cloud.
- Aging data infrastructures and legacy architectures directly impact AI/ML’s sustainability performance. More than three-fourths (77%) of respondents believe data architectures impact their sustainability performance.
- Organizations that have their data and infrastructure “houses” in order will be well-positioned to lead with AI in the future. Companies leveraging a modern data architecture to overcome significant data challenges (sources, types, requirements, etc.) can accommodate AI/ML workloads operating across multiple infrastructure venues.
Organizations should heed the findings of the 2023 Global Trends in AI Report, leverage the data-driven insights as a benchmark, and contextualize the results within their organization’s unique circumstances to guide their AI strategy in 2023 and beyond.
AI is rapidly maturing at enterprise scale, impacting business outcomes
AI projects and use cases have largely passed a tipping point within enterprises, with most survey respondents (69%) having transitioned at least one AI deployment to a production environment. A more detailed characterization of AI adoption comes with measuring its impact across the entire enterprise, which could include multiple AI projects, use cases and deployments driving current and future business strategies.
Figure 1: The pulse of AI: maturing projects and enterprise scale
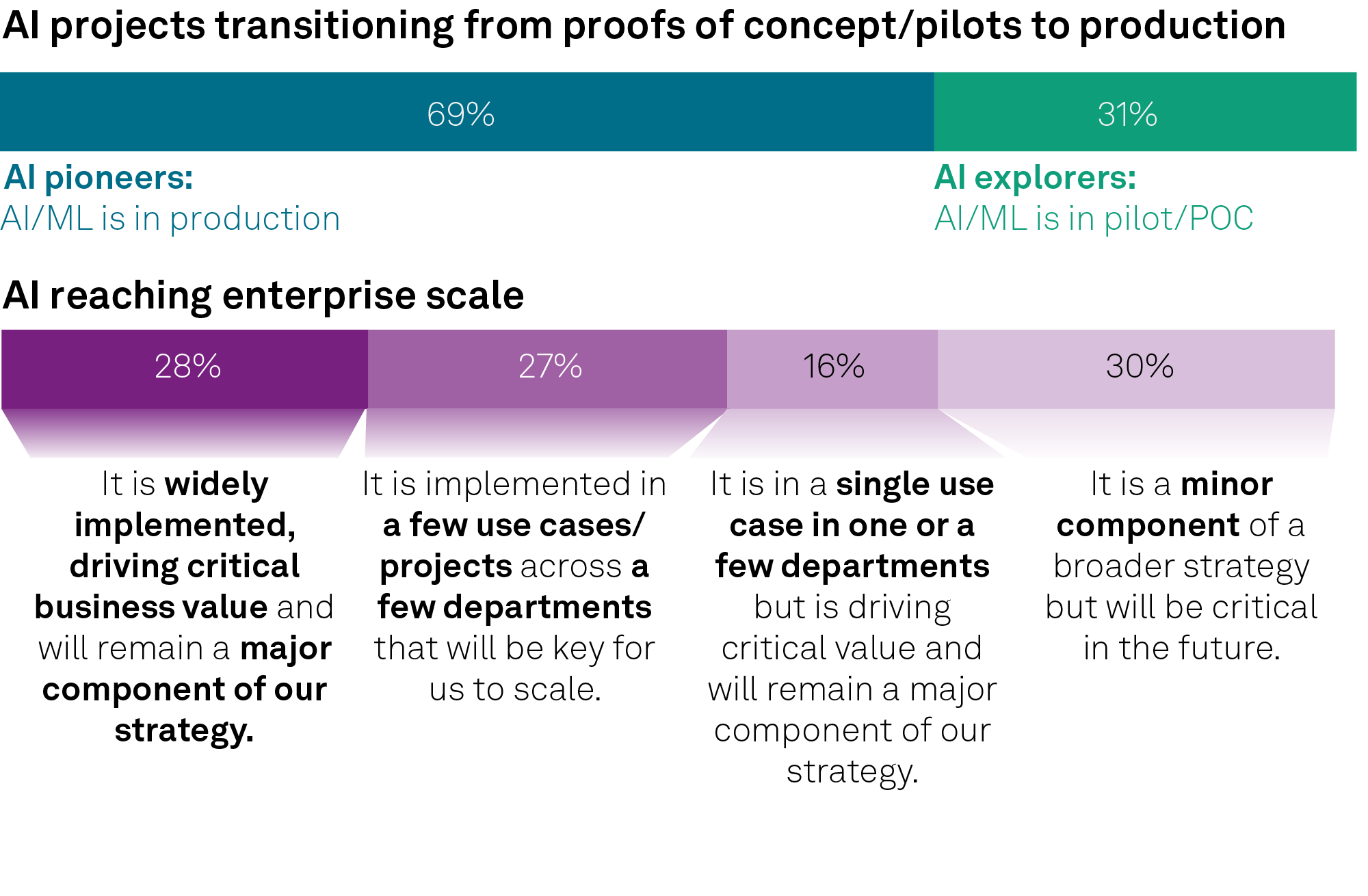
- Q. Which of the following best describes your organization’s current AI/ML adoption status?
- Q. How would you best describe the criticality of AI/ML to your current and future strategy?
- Base: All respondents (n=1,516).
- Source: 451 Research’s 2023 Global Trends in AI custom survey.
Current adoption in enterprises aligns with differences in the scale of AI projects. Some (28%) have widely implemented AI across their business, while others (30%) say it is still a minor component. Regardless, for most organizations, it is clear that use of AI is no longer restricted to a small pool of IT or data science specialists but is rather a cross-functional capability used by an array of line-of-business teams. We expect that organizations will continue to expand AI’s role in their strategies, as 94% of respondents to a recent 451 Research Voice of the Enterprise survey1 indicated that AI is important to their organization’s digital transformation efforts.
Figure 2: Revenue drivers lead cost drivers for AI/ML
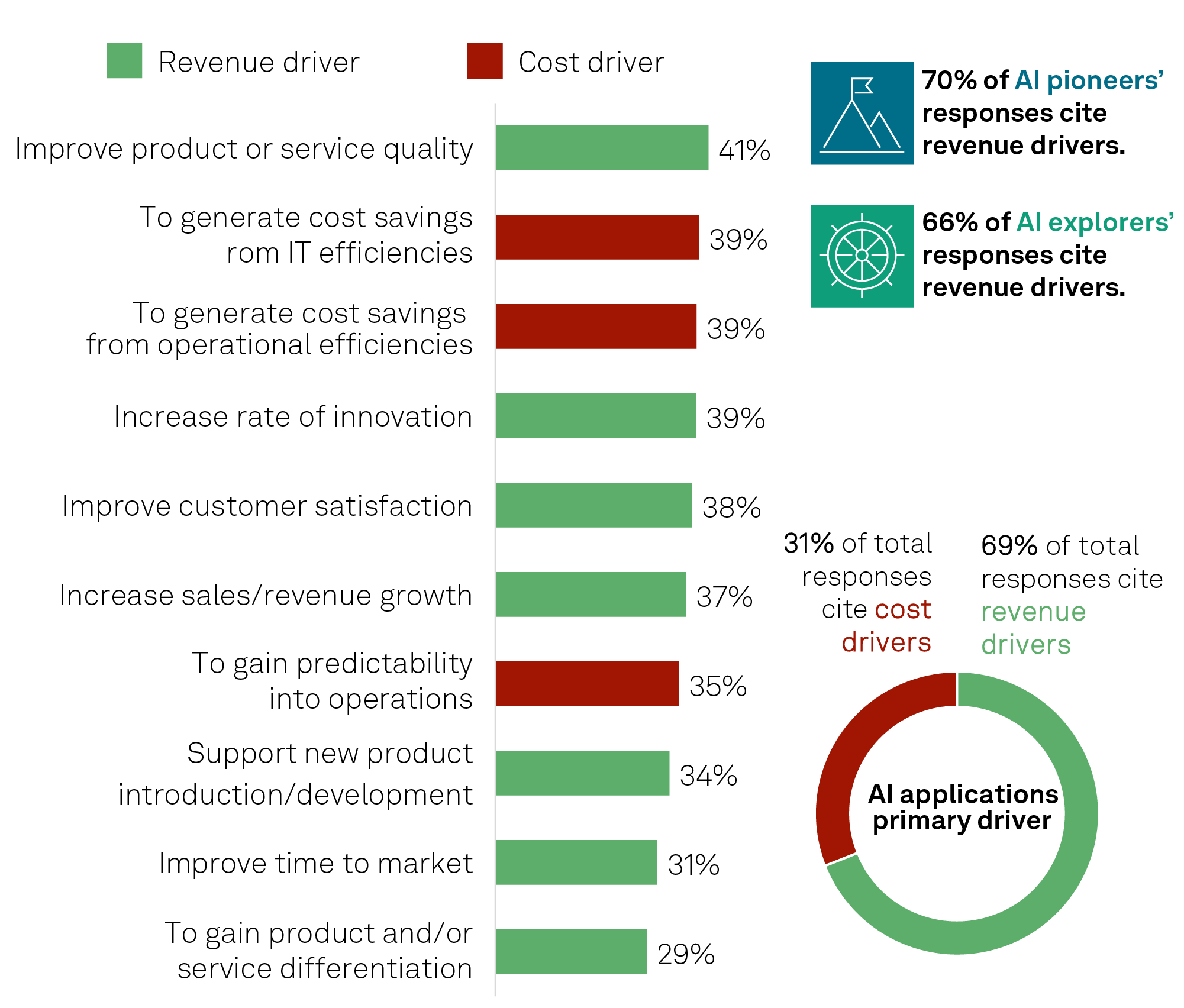
- Q. What are the primary driver(s) for developing AI/ML applications at your organization?
- Base: All respondents (n=1,516; 5,478 total responses).
- Source: 451 Research’s 2023 Global Trends in AI custom survey.
While enterprises can unquestionably extract greater value from their data via better data management and optimization of their infrastructure, they are putting their AI/ML deployments to good use today. As with many other automation technologies, the value of AI has, in many instances, been tied to cost reduction. Survey respondents depict a much more multifaceted value proposition, with AI/ML directly driving top-line growth – opening new revenue streams, driving sales initiatives and enhancing product portfolios and services.
Organizations are affecting their top line with AI by improving product and service quality, ensuring customers are achieving the quality and outcomes they expect from products and services they’ve purchased. AI-enabled tools such as generative design help product engineers increase the rate of innovation and accelerate new product introduction/development processes. Generative AI use cases are the latest revenue drivers, such as increasing sales reach with mass personalization of communication enabling direct interface with customers or providing prescriptive recommendations, among countless others.
Organizations’ IT infrastructure and data architectures are unfit for the AI revolution
Enterprise IT and data architectures are straining under the weight of ever-expanding AI workloads. Industry attention has centered on the cost and availability of GPUs, but survey respondents present a much wider-ranging set of bottlenecks, most closely tied to data management. Data is the lifeblood of AI, but if left unchecked, it can quickly become a deterrent. Larger organizations with more than 10,000 employees struggle to get their arms around the enormous volumes of data required to train AI models. As organizations set themselves up to holistically address data management, additional challenges arise from an increasingly complex ecosystem of deployment locations, use cases and stakeholders.
Figure 3: Data management is the top infrastructure-related challenge to AI efforts
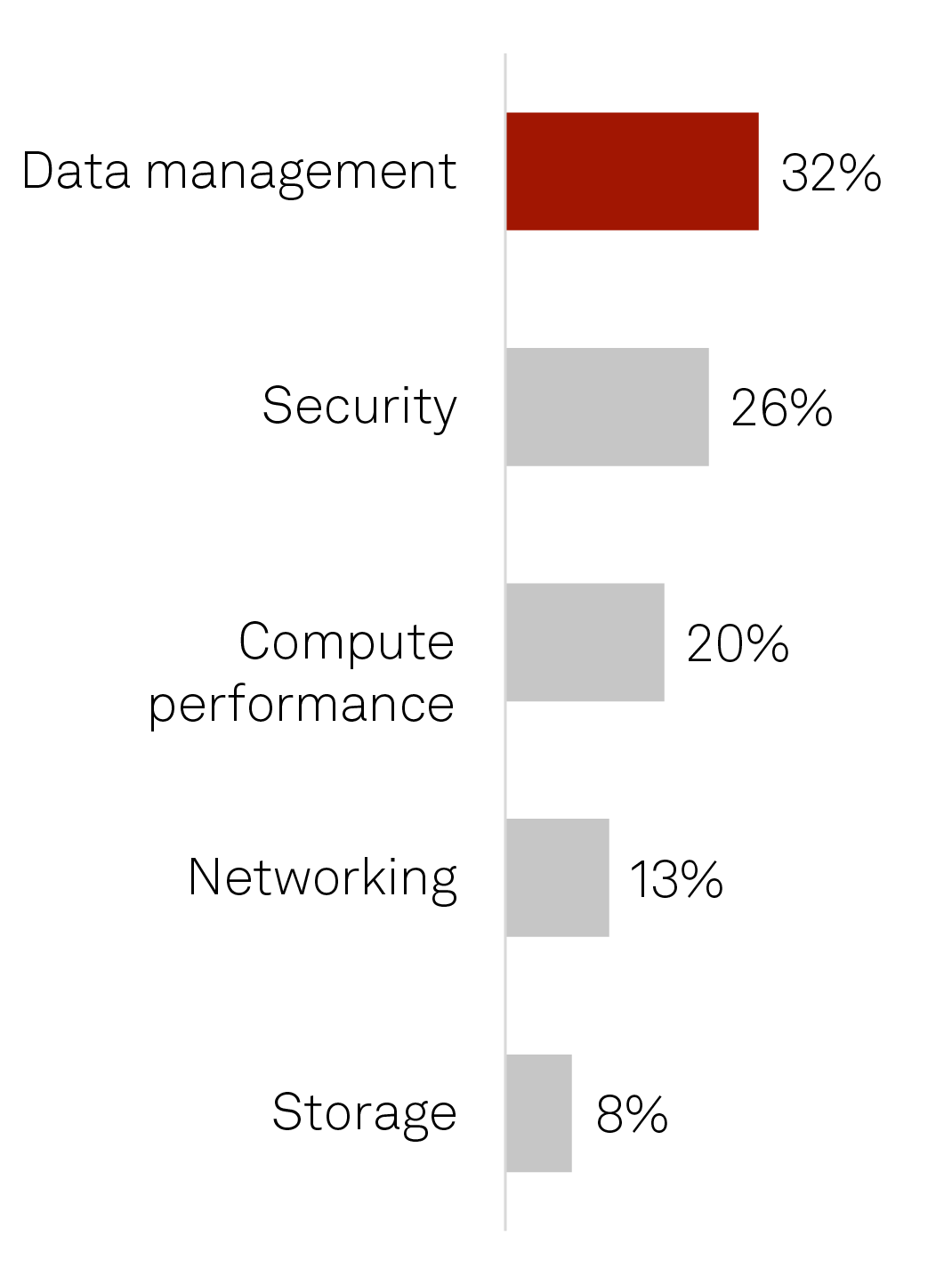
- Data management top challenge: Outweighs other important infrastructure components including security, and compute performance.
- Across AI/ML applications life cycle: Data sourcing, preparing, standardizing, training/inferencing a model and testing/deploying a trained model all pose unique and collective challenges.
- POC to production: Moving from pilot to production is slowed down by developing/implementing inferenced models and legacy architecture cannot support AI/ML applications.
- Data governance grumblings: Skillset gaps, data control and data silos cause compounding data governance challenges.
- Data structure standardization: Burdened by insufficient IT infrastructure and unwieldy data volumes.
- Different data types: Unique requirements for structured data, unstructured text and streaming/real-time data.
- Q. What technological component of your infrastructure is the greatest inhibitor to your AI/ML application deployments?
- Base: All respondents (n=1,516).
- Source: 451 Research’s 2023 Global Trends in AI custom survey.
The challenges start with the fact that data for training AI models can come in many types. These include structured data such as databases, used by 66% of respondents, and streaming/real-time data (52%) such as IoT telemetry data across a fleet of construction equipment. IT system data, including log files (51%) and semi-structured data types such as email (43%) can be leveraged for critical IT AI applications in IT Ops or cybersecurity. Training models on unstructured rich media data (37%), including images, is increasingly required for computer vision applications, and unstructured text (35%) is quickly becoming a top data source for generative AI applications. Maintaining data standardization is a challenge when considering this multitude of data types. Further complicating matters, many enterprises have insufficient IT infrastructure (cited by 41% of respondents), unwieldy data volumes (50%) and a lack of internal expertise (38%). Managing data governance to ensure data quality and compliance is also hampered by a lack of internal skillsets (39%) and data control (37%).
Once an organization has overcome these significant data challenges and developed a well-functioning POC, it still may face challenges in transitioning to production environments that were built for traditional data-driven applications, including retraining AI models, achieving optimal performance or scaling infrastructure to meet the required volume of data.
AI and modern infrastructure provide a path to sustainability despite near-term costs
The wearied mantras “data is the new oil” and “data is the new gold” have transitioned to “data is the new green”: enterprises are working to monetize their latent troves of data. AI is a catalyst to unlock the treasures in these vast amounts of data, to create new revenue streams or drive significant efficiencies and reduce costs.
But cost savings and more dollars are not the only “green” future AI can provide. AI can also contribute to sustainability initiatives, such as by leveraging AI to build eco-efficient products (i.e., generative design), optimize supply chain activities (transportation, sourcing parts, etc.), and monitor and reduce energy consumption. Most survey respondents say these AI-enabled use cases drive medium to high impact on sustainability performance today.
Figure 4: AI is driving sustainability use cases
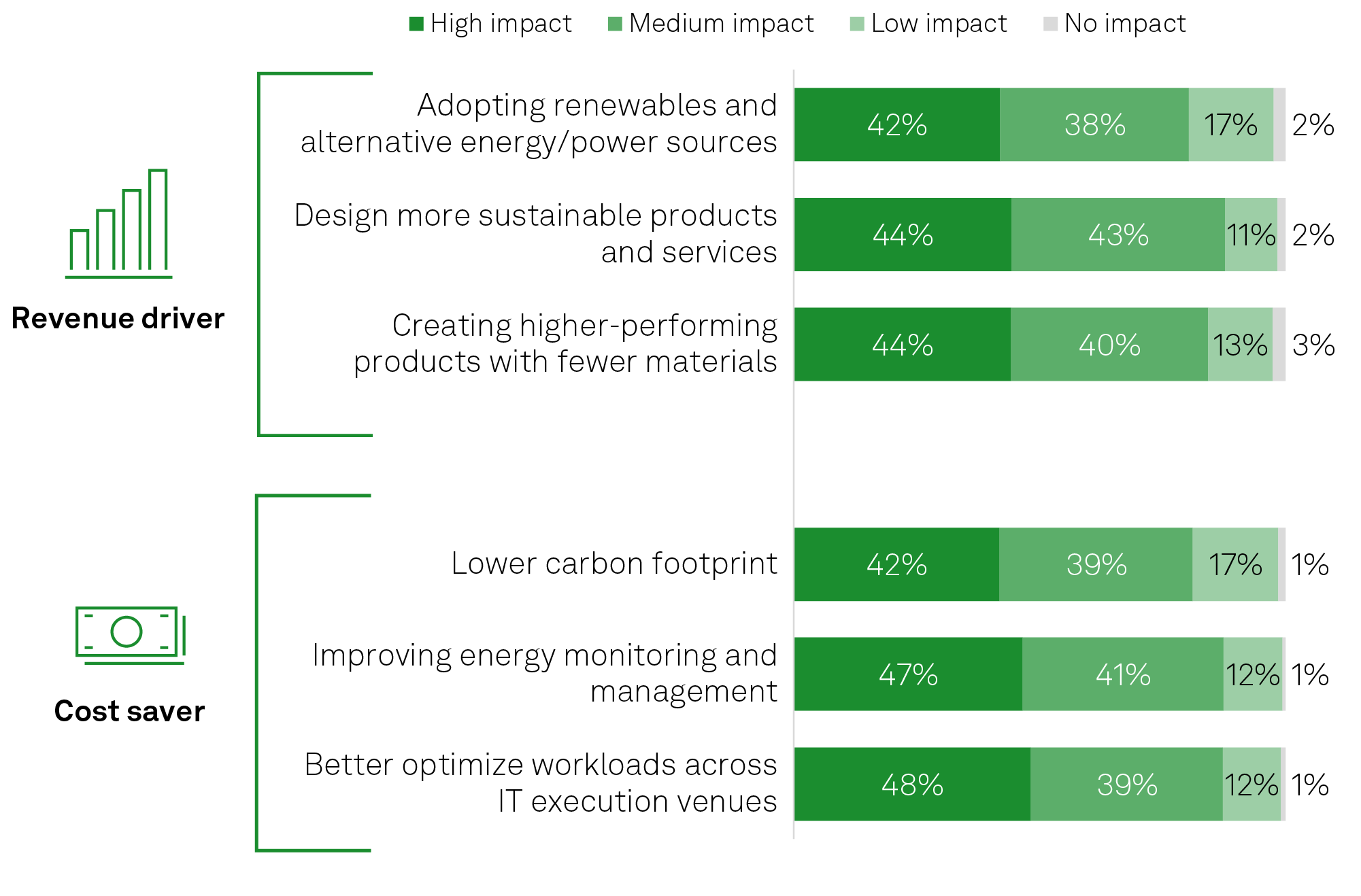
- Q. For each of the following, what has been the impact of AI on sustainability performance?
- Base: Respondents who report that AI/ML has impacted their sustainability performance (n=610).
- Source: 451 Research’s 2023 Global Trends in AI custom survey.
Organizations are leveraging these sustainability-friendly use cases to drive corporate sustainability goals (CSGs). There are some minor differences in CSG program maturity, with 31% of AI pioneers executing on a formal program driving significant impact compared to 28% of AI explorers. Additionally, there are regional nuances: North America (34%) has the greatest proportion of respondents in the CSG execution stage, followed by Asia-Pacific (29%) and EMEA (28%).
Figure 5: Organizations taking multiple steps toward corporate sustainability goals
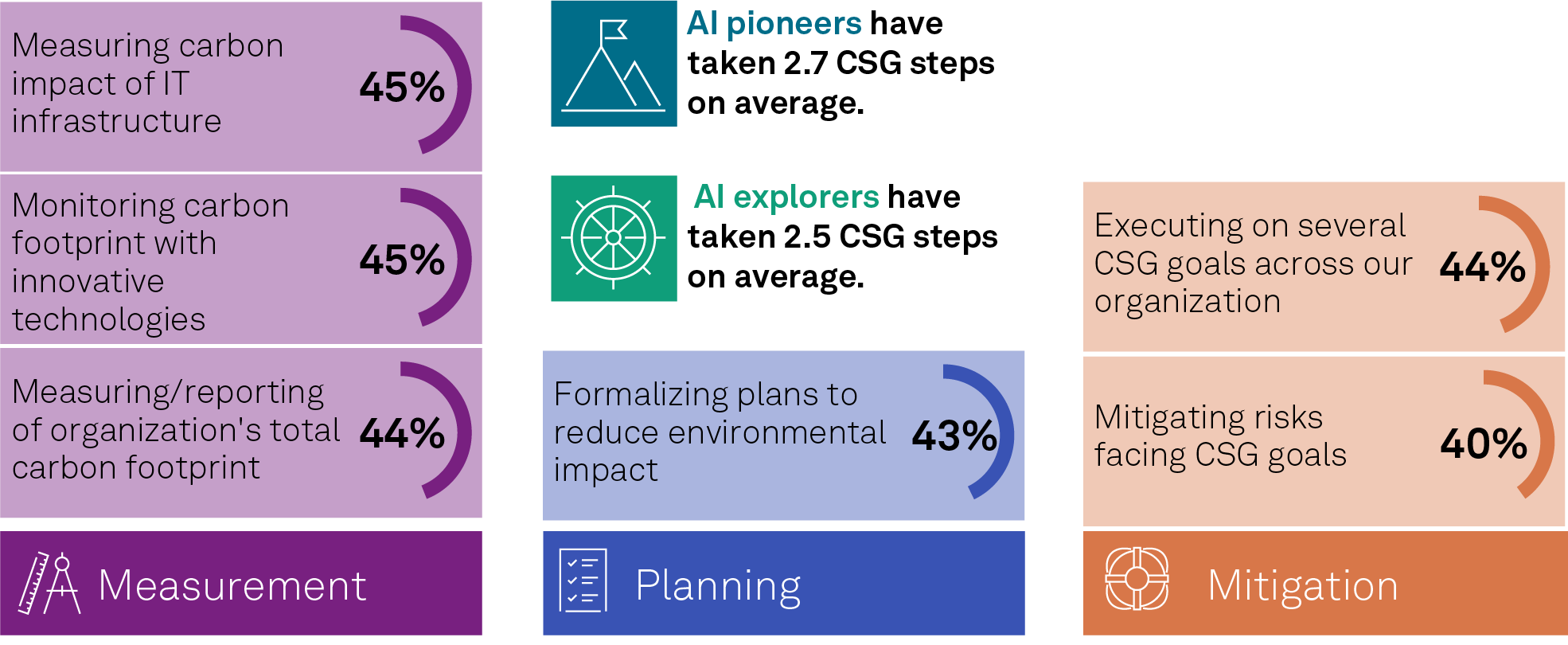
- Q. What steps has your organization taken to reach its corporate sustainability goals (CSGs)?
- Base: All respondents (n=1,516).
- Source: 451 Research’s 2023 Global Trends in AI custom survey.
While organizations are quickly maturing their CSG programs by using powerful yet data-intensive AI/ML technologies to unlock green solutions, processing vast amounts of data also has environmental consequences: training GPT-3, the neural network model underlying Chat GPT, was estimated to exceed 500 billion tons of carbon emissions. 1 The toll on infrastructure to train AI models is significant when considering the power to support datacenter racks, water and HVAC systems to cool them, the real-time data processing and storage at scale, among other system requirements. Considering these efficiency challenges, impending regulatory frameworks governing organizations’ carbon emissions and the overall demands of scaling up these systems to train AI, the balancing point between AI’s costs and its potential positive impacts can become foggy.
Fortunately, AI is also a means to improve infrastructure by allocating resources more efficiently, optimizing workloads through automated provisioning and improved performance, and automating IT tasks. The public cloud is bringing clarity to AI, where AI pioneers have recognized its impact via sustainability improvements; 40% of AI pioneers say sustainability is a critical driver for moving more workloads to public cloud infrastructure versus 25% of AI explorers.
The modernization of infrastructure and its impact on sustainability expands to high-performance computing (HPC): 84% of respondents cite HPC as critical/very important and a requirement for AI/ML applications. Additionally, 53% of those with AI widely implemented in their organization today say data architectures significantly impact sustainability performance compared to 27% of those with only a few AI projects deployed.
The takeaway is that as AI deployments scale, non-optimized IT infrastructure is a growth bottleneck, and the impacts on sustainability can be severe. Getting infrastructure positioned for AI growth is also important to lessen the top two sustainability challenges: the high costs to transition to more efficient systems (46% of respondents) and initiate programs (45%).
It will be critical for AI leaders to view initial investments in modernizing their IT and data infrastructures as necessary to support future growth and make good on projected costs or ROI claims in sustainability programs.
Enterprises with their data/infrastructure houses in order are poised to be future AI leaders
In its simplest form, a modern data architecture maintains the data life cycle from its initial source through processing, analytics (AI/ML) and storage (databases, data lakes). Due to a myriad of legacy processes, databases, infrastructure and systems, enterprises are turning to the public cloud as an easier lift to implement a modern data architecture. With data-intensive and complex AI applications, the public cloud is a common starting place and is the top primary deployment model for training AI/ML models (47%) and inferencing (44%).
While the public cloud is unquestionably critical to developing and deploying AI/ML models, their life cycle requires processing at multiple deployment locations requiring a hybrid approach. This is especially true for AI pioneers with AI/ML in production, who are more likely to use multiple training and deployment locations versus AI explorers with AI/ML in pilot/POC. Specifically, AI pioneers on average use 3.2 deployment locations for training and 2.5 for inference, versus 2.9 and 2.3, respectively, for AI explorers.
Figure 6: AI/ML pioneers optimize their entire infrastructure
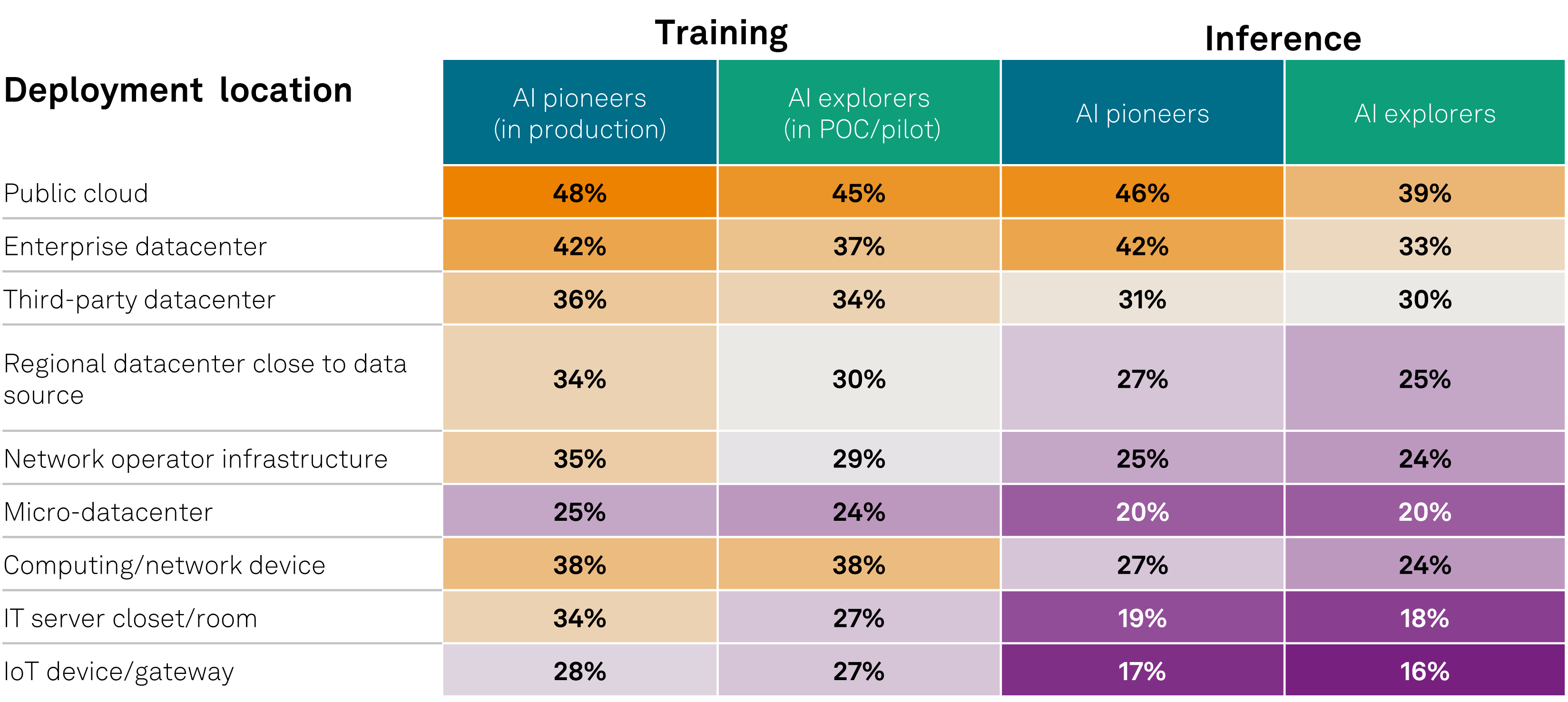
- Q. Where is the primary execution venue for training AI/ML models?
- Q. Where is the primary execution venue for inference from trained AI/ML models?
- Base: All respondents (n=1,516).
- Source: 451 Research’s 2023 Global Trends in AI custom survey.
This can be attributed to a few coinciding trends. First, AI/ML workloads have a natural gravitational pull toward multiple deployment locations depending on their use case and point in the life cycle. For example, an autonomous vehicle could use some AI/ML inference at its point of origin (device/gateway) to navigate driving environments, then at a network operator infrastructure to optimize routes across a fleet, and then again at the public cloud for analysis of rider history or vehicle performance. With AI/ML’s ability to revolutionize edge computing and vice versa, the symbiotic relationship can be fundamental to unlocking next-generation data-intensive AI use cases such as computer vision, AR/VR, robotics and others. Edge capabilities will, in turn, benefit AI/ML applications with pre-processing, regulatory compliance, network resiliency and latency sensitivity.
Second, those who have scaled AI use cases are not constrained by the limitations of a legacy data architecture and pigeonholed into a single deployment location, whereas many in POCs/pilots may only be running an AI application in one or two locations for simplicity. However, as mentioned, AI/ML naturally operates across multiple locations when in production. Maintaining the hygiene of data architecture to facilitate seamless data exchanges between locations is ultimately a necessity to scale AI in production.
Additionally, AI pioneers are more likely to have optimized their infrastructure via high-performance computing; 50% of AI pioneers cite HPC as critically important and a fundamental requirement for AI/ML applications versus 30% of AI explorers. Those who have formed a modern data architecture and have their data and infrastructure “houses” in order are not dismayed by the demands of a data life cycle spanning multiple locations — they can efficiently manage the AI/ML data life cycle across venues and drive AI project success.
The value derived from AI varies by region, industry and size
The value that organizations report from their AI investments varies by company size, region and industry. When looking at the challenges companies face in scaling AI, size is a notable differentiator — in some instances, the larger the company, the more challenging AI projects become. Larger enterprises tend to leverage their extensive resources to propel technology projects, yet they can face substantial challenges from legacy systems, change management and stakeholder buy-in.
While only 58% of organizations with revenue of $100 million-$499 million have AI in production, that jumps to 82% of those with $500 million-$5 billion in revenue, then falls to 76% of those with $5 billion+ revenue. AI’s regional impact differs based on geopolitical forces, support of free markets, enforcement of regulatory environments and access to talent, among other factors.
Figure 7: AI maturity benchmarks — company revenue, region and industry
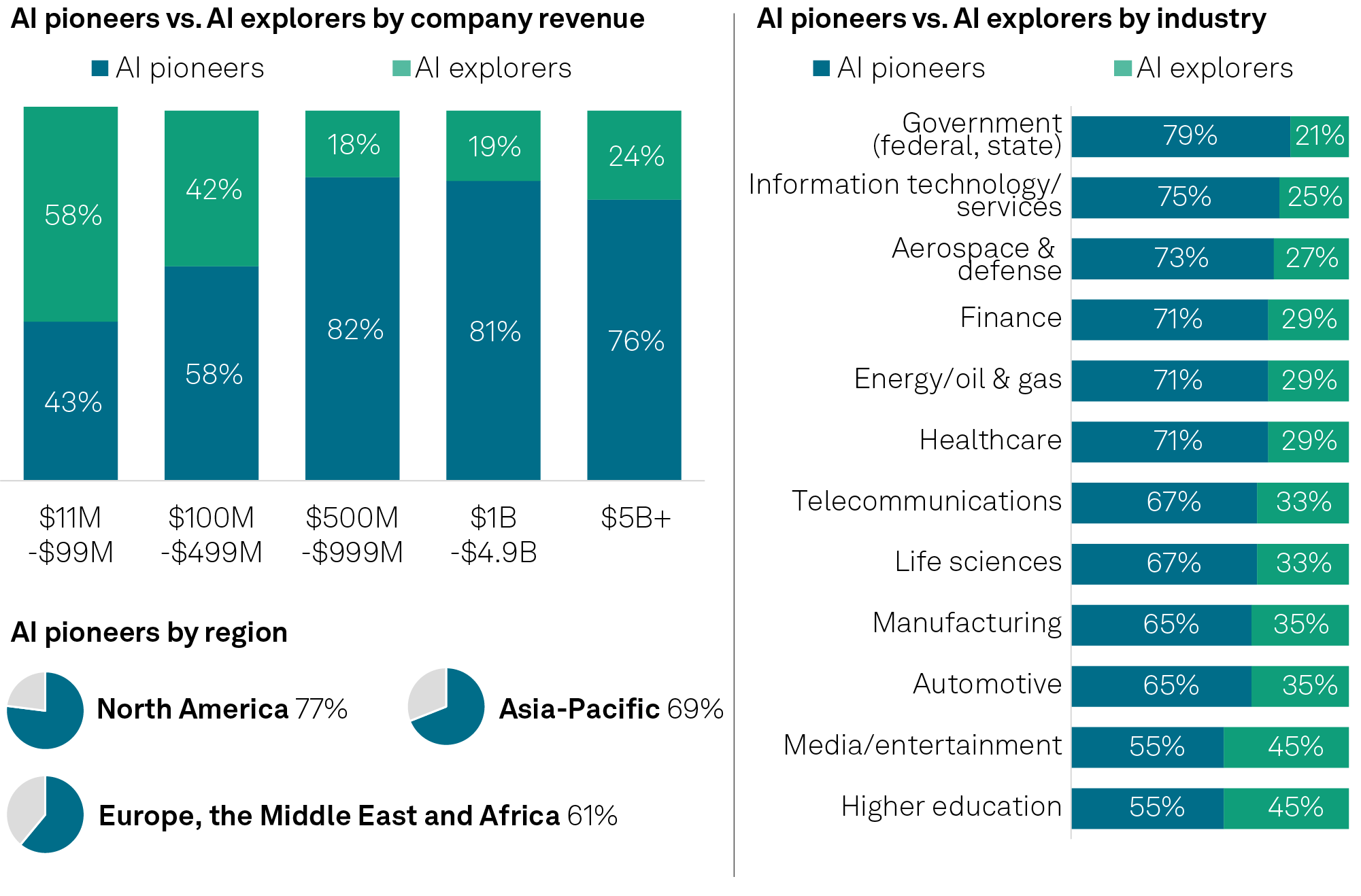
- Q. Which of the following best describes your organization’s current AI/ML adoption status?
- Base: All respondents (n=1,516).
- Source: 451 Research’s 2023 Global Trends in AI custom survey.
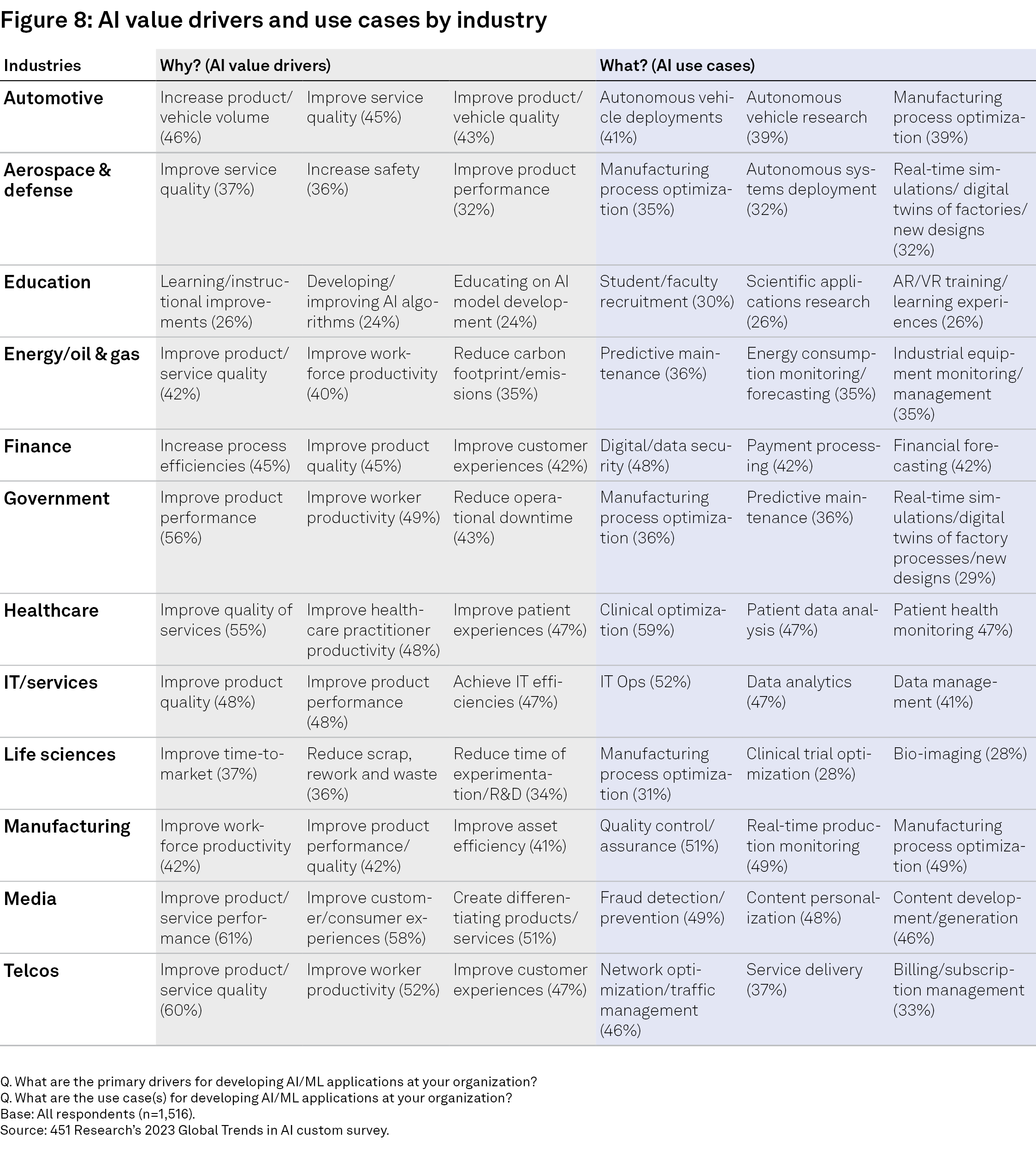
Across every industry, value drivers or business outcomes are prioritized as top areas of intiative to improve an organization’s competitive standing and optimize operations. For example, with a formidable skills gap and workforce shortage looming in the manufacturing sector, many manufacturers are prioritizing workplace productivity improvements. In the healthcare industry, where patient satisfaction is critical, organizations position their quality of services and patient outcomes as differentiators. These value drivers (the “why”) are the business outcomes that AI/ML impacts across industries, while innovative use cases (the “what”) leverage AI/ML-enabling technologies to unlock the desired value.
Aerospace & defense
Aerospace & defense gravitates toward ensuring the quality and consistent performance of their airplanes, drones, UAVs and space systems to support stringent safety compliance requirements. This emphasis on quality and safety starts with a laser focus on product development and the manufacturing process, where digital twins run complex “what if” simulations to predict outcomes in factories. AI/ML’s ability to rapidly ingest vast amounts of image data supports computer vision-inference models that make mission-critical instantaneous decisions on autonomous systems such as drones or automated vehicles.
Automotive
Automakers continue to prioritize improving the rate at which they can design, build, ship and bring new vehicles to market and increase the overall production volume of new vehicles. To support this, they are looking to AI to optimize manufacturing processes and more accurately predict patterns from operational data and industrial systems. This predictability enables automakers to improve overall equipment effectiveness (OEE), throughput, product quality and other manufacturing efficiency metrics. Given the substantial data volume and processing requirements, it is not surprising that autonomous vehicle research and deployment are the top AI use cases for automotive; on-car data generation from assisted/autonomous driving features is projected to increase from 4 billion GBs in 2022 to 50 billion GBs in 2030.1
Education
Educators are turning to AI as an instructional tool, a method for advanced forms of research, and a means to equip students with the knowledge to develop models and acquire data science skills. Administrators are also leveraging AI in recruiting students and faculty by sorting through data including skillsets, experiences, certifications and other qualifications. Many universities fund and develop AI accelerators to support research and discovery projects in robotics, medicine, public health, life and environmental sciences, and more.
Energy/oil & gas
Energy and oil and gas companies focus on improving the quality of their products and services, and increasing worker productivity through AI. To support these outcomes, they cite predictive maintenance and process optimization as their top two AI use cases. AI can also positively affect sustainability efforts, and leveraging AI to reduce carbon footprint is ranked highly among those in the energy sector.
Financial services
Nearly half of financial services respondents report investing in digital and data security. Alongside payment processing and financial forecasting, fraud detection and prevention is a focal point for many businesses. As a tool for pattern recognition, AI is hugely valuable in improving the accuracy of triaging fraudulent accounts and payment transactions, and it is increasingly tied into low-latency and data-intensive applications, including algorithmic and high-frequency trading.
Government
Regulatory environments are pushing national and local governments to rapidly develop AI/ML capabilities. That can include optimization efforts such as traffic flow analysis to relieve congestion or predictive maintenance to better predict downtime on critical assets such as infrastructure.
Life sciences
Pharmaceutical companies put importance on maximizing their manufacturing capacity to fulfill demand. In addition to continuous manufacturing activities, AI can drive value by accelerating drug development in R&D and fulfilling laborious compliance processes, which can speed up time to market. Further, bio-imaging is an emerging non-invasive technique to more accurately identify diseases and other abnormalities. AI models can ingest volumes of health and patient data (i.e., records, images) and use these to inform predictions and potential diagnoses.
Media & entertainment
Content recommendations and personalization can benefit from AI, and this role has evolved from merely extracting patterns in customer data to algorithmic curation and even — with generative AI — personalized content creation. These advancements in content development and personalization are key to media & entertainment companies improving consumer experiences and differentiating products and services.
Conclusions
The 2023 Global Trends in AI Report reflects a mix of emerging and ongoing innovations driving an unprecedented pace of innovation. A significant proportion of organizations — with some nuances in regions of operation, industry and size — are better prepared and positioned to accommodate this influx of AI innovation.
Many in this “AI pioneer” category have optimized their infrastructure and gotten their data “houses” in order, providing a foundation for developing new AI use cases and scaling existing ones. Organizations with this sound data setting will view AI as an opportunity to drive sustainability and new business value rather than as a cost center.
- AI is no longer something futuristic to prepare for — now is the time to harness its value. Companies still formulating a strategy to prepare for AI may need to accelerate their plans. AI is quickly becoming a revenue driver, and organizations must begin executing a strategy to propel long-term growth.
- Sustainability should not be overlooked in favor of focusing on short-term costs. Cost and sustainability should not be treated as competing trade-offs in selection criteria. Doing so neglects the longer-term alignment between the two objectives. AI can be critical in improving efficiencies in IT architecture and across operations and supply chains, and in driving sustainable business models and use cases. Investments in modernizing data architectures could reduce an organization’s long-term energy use and environmental impact.
- Prepare for AI infrastructure and data strategies to broaden and scale. As AI initiatives shift from pilots and proofs of concept to in-production and at scale, organizations need to plan for an array of data and infrastructure environments for data preparation, training and inference, including variations required to address diverse use cases. While many projects start in the cloud, factors such as latency and data sovereignty can drive organizations to invest in data architectures supporting venues from the core to the edge. Fostering a “prepare to scale” mindset from the AI project’s inception puts the proper investments in place to seamlessly facilitate enterprise-wide adoption.
Companies have an opportunity to reinvent their value proposition for AI to drive revenue or revitalize their operations to improve profits. Every company must navigate its own path to lead with AI.
Methodology
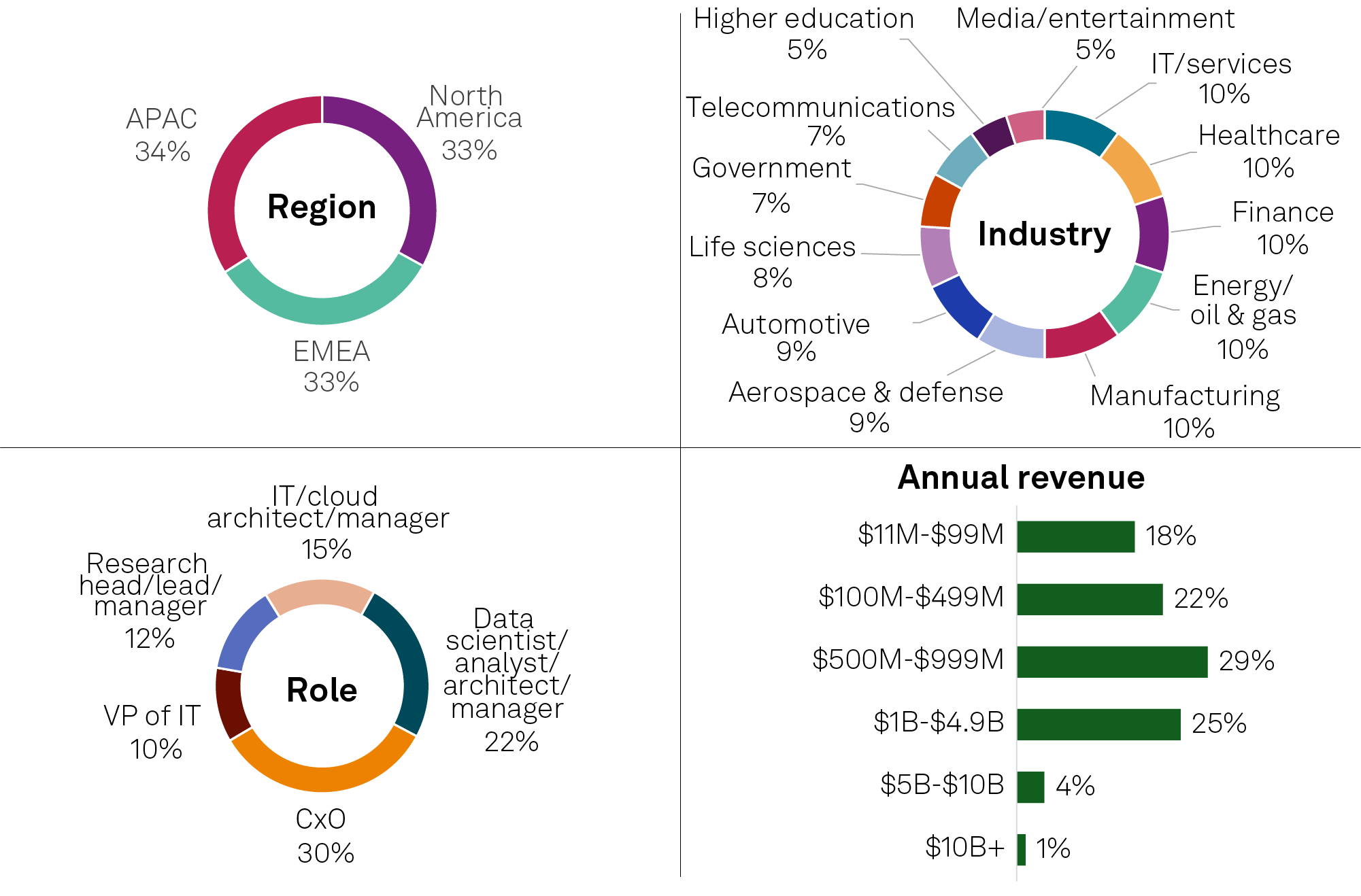
The findings presented in this report draw on a survey fielded in North America, Europe, the Middle East, Africa and Asia-Pacific in Q2 2023.
The survey targeted 1,516 AI/ML decision-makers/influencers in companies with over 250 employees and over $10 million in annual revenue. The study prioritized respondents with AI/ML deployed in pilots and production environments across the following industries: aerospace & defense, automotive, higher education, finance, energy/oil & gas, government, healthcare, IT/services, life sciences, manufacturing, media/entertainment and telecommunications. The leading job descriptions of respondents were data scientist, analytics and architect. This report also draws on contextual knowledge of additional research conducted by S&P Global Market Intelligence.
About the authors
About this report
A Discovery report is a study based on primary research survey data that assesses the market dynamics of a key enterprise technology segment through the lens of the “on the ground” experience and opinions of real practitioners — what they are doing, and why they are doing it.
About S&P Global Market Intelligence
S&P Global Market Intelligence’s Technology, Media and Telecommunications (TMT) Research provides essential insight into the pace and extent of digital transformation across the global TMT landscape. Through the 451 Research and Kagan products, TMT Research offers differentiated insight and data on adoption, innovation and disruption across the telecom, media and technology markets, backed by a global team of industry experts, and delivered via a range of syndicated research, consulting and go-to-market services, and live events.
CONTACTS
Americas: +1 800 447 2273
Japan: +81 3 6262 1887
Asia Pacific: +60 4 291 3600
Europe, Middle East, Africa: +44 (0) 134 432 8300
www.spglobal.com/marketintelligence
www.spglobal.com/en/enterprise/about/contact-us.html
Copyright © 2023 by S&P Global Market Intelligence, a division of S&P Global Inc. All rights reserved.
These materials have been prepared solely for information purposes based upon information generally available to the public and from sources believed to be reliable. No content (including index data, ratings, credit-related analyses and data, research, model, software or other application or output therefrom) or any part thereof (Content) may be modified, reverse engineered, reproduced or distributed in any form by any means, or stored in a database or retrieval system, without the prior written permission of S&P Global Market Intelligence or its affiliates (collectively S&P Global). The Content shall not be used for any unlawful or unauthorized purposes. S&P Global and any third-party providers (collectively S&P Global Parties) do not guarantee the accuracy, completeness, timeliness or availability of the Content. S&P Global Parties are not responsible for any errors or omissions, regardless of the cause, for the results obtained from the use of the Content. THE CONTENT IS PROVIDED ON “AS IS” BASIS. S&P GLOBAL PARTIES DISCLAIM ANY AND ALL EXPRESS OR IMPLIED WARRANTIES, INCLUDING, BUT NOT LIMITED TO, ANY WARRANTIES OF MERCHANTABILITY OR FITNESS FOR A PARTICULAR PURPOSE OR USE, FREEDOM FROM BUGS, SOFTWARE ERRORS OR DEFECTS, THAT THE CONTENT’S FUNCTIONING WILL BE UNINTERRUPTED OR THAT THE CONTENT WILL OPERATE WITH ANY SOFTWARE OR HARDWARE CONFIGURATION. In no event shall S&P Global Parties be liable to any party for any direct, indirect, incidental, exemplary, compensatory, punitive, special or consequential damages, costs, expenses, legal fees, or losses (including, without limitation, lost income or lost profits and opportunity costs or losses caused by negligence) in connection with any use of the Content even if advised of the possibility of such damages.
S&P Global Market Intelligence’s opinions, quotes and credit-related and other analyses are statements of opinion as of the date they are expressed and not statements of fact or recommendations to purchase, hold, or sell any securities or to make any investment decisions, and do not address the suitability of any security. S&P Global Market Intelligence may provide index data. Direct investment in an index is not possible. Exposure to an asset class represented by an index is available through investable instruments based on that index. S&P Global Market Intelligence assumes no obligation to update the Content following publication in any form or format. The Content should not be relied on and is not a substitute for the skill, judgment and experience of the user, its management, employees, advisors and/or clients when making investment and other business decisions. S&P Global keeps certain activities of its divisions separate from each other to preserve the independence and objectivity of their respective activities. As a result, certain divisions of S&P Global may have information that is not available to other S&P Global divisions. S&P Global has established policies and procedures to maintain the confidentiality of certain nonpublic information received in connection with each analytical process.
S&P Global may receive compensation for its ratings and certain analyses, normally from issuers or underwriters of securities or from obligors. S&P Global reserves the right to disseminate its opinions and analyses. S&P Global’s public ratings and analyses are made available on its websites, www.standardandpoors.com (free of charge) and www.ratingsdirect.com (subscription), and may be distributed through other means, including via S&P Global publications and third-party redistributors. Additional information about our ratings fees is available at www.standardandpoors.com/usratingsfees.