Life Science Data Management [Everything You Need to Know]
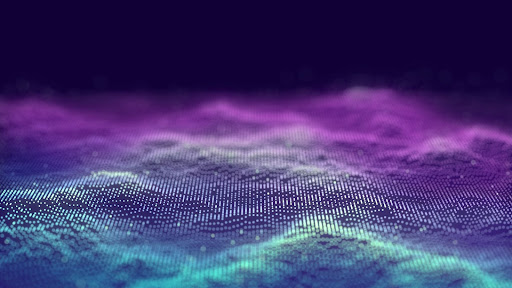
Are you interested in life science data management? We explain what it is, the solutions you need, and best practices for managing your data.
What is life science data management? Life sciences generate massive amounts of data used for research such as disease identification, drug discovery, clinical trials, and genome sequencing. Life science data management is the practice of collecting, storing and processing all that data.
What Is Life Science Data and What Does It Mean to Manage It?
Data is the lifeblood of operations in healthcare. Pharmaceuticals, genomic sequencing, and on-the-ground health providers are all leaning on big data platforms to help fuel their research, diagnostics, manufacturing, and customer service.
This opportunity to use big data as a research and production tool comes with a few significant challenges. Healthcare data is incredibly sensitive, and as such, companies handling it must consider a few issues:
- Security and Compliance: Protected health information is one of the most heavily regulated forms of private data globally. Organizations managing data, whether for research or just day-to-day treatment, must consider how they are securing that information based on HIPAA regulations.
- Data Silos: Pulling data from various disparate sources can choke a program’s progress before it can get started. Life sciences aren’t different in that regard, as they often pull information from several different sources to feed an analytics or machine learning platform.
- Structure and Accuracy: Even integrating data has its issues. Unvetted, unstructured data from various sources can be error prone, unclassified, etc. In life sciences research, data accuracy is critical, and not having that accuracy can derail the success of a project.
- Good Practice (GxP): GxP refers to quality standards and regulations for pharmaceuticals and life sciences. If your life sciences project relates to developing medical equipment or drugs, then you’ll have to meet specific data management and security guidelines.
Life sciences data management is rooted in concerns of compliance and security, data governance, collaboration, and accurate automation and processing.
What Is a Life Sciences Data Management Strategy?
With the vast quantity of data managed in life sciences, it isn’t sufficient to deploy data platforms and processing applications without thinking about how that data will move through your system.
Consider that, during the life cycle of a research project, only part of the data will be directly processed for sequencing a genome or providing critical analytics. Most projects will also include tertiary data produced during the process, including documentation and reports, instrument diagnostics, operational data for the system, and administrative paper trails.
It’s critical, then, that your organization has a data management strategy for your life science data management.
One way to approach such a strategy is to use the FAIR model:
- Findable: Refers to how easy it is to find or search for data, which is more complex than one might think. Admins wrestling with accessibility must consider folder arrangement and relevance to the project, logical naming nomenclature for files and folders, and maintaining registries. It also refers to aspects of data management like organizing and maintaining metadata to keep data searchable.
- Accessible: Slightly different from findability, accessibility is the assurance that relevant people and project resources should access the data specific to their role in the project. Sensitive data should be separately cataloged and maintained by a data manager, but it shouldn’t be isolated from a project due to its sensitivity. There should also be controls to facilitate the sharing of data between internal and external stakeholders.
- Interoperable: Make sure that data is usable and transferable across systems, people, and departments. This means using templates for easy document creation and sharing, deploying usable file formats that everyone can read (and that machines can ingest, if necessary), and fitting these templates and documents into your workflow.
- Reusable: Create, maintain, and expand metadata as needed so that information can move from one context to the next and have controls in place to ensure that the data life cycle extends beyond the project’s immediate needs or a particular part of the project.
What Is a Data Steward?
Part of effective data management strategies is having people in the right roles in the right place. However, something that comes up in many discussions of cloud-based life science research is having a data steward.
What, exactly, is a data steward? This person is a domain expert who steps into the role of managing the critical questions around data in a project. For example, a data steward might step in to ensure that data remains well-organized, findable, and accessible.
Data stewards can cover areas such as the following:
- Research: These data stewards will often help their colleagues navigate complex cloud, high-performance computing or data usage. These people serve as intermediaries between the technology and the people and serve a critical role in driving the project daily.
- Infrastructure: These stewards actually help build the tools used for these projects. For example, this steward may develop specialized software to help process data, like software used by their colleagues to help them manipulate or make sense of that data.
- Policy: An organization will often rely on one or more people to serve as points of communication for questions of policy, procedure, or compliance. Some jurisdictions, like the EU and GDPR, require it.
What Should I Look for in a Solution?
Regardless of how you steward data or strategize management, your solution must cover specific areas with specific controls. Some of the primary aspects of a management solution that you should consider include the following:
- Security and Compliance Controls to meet industry needs.
- Fast, High-Volume Storage for massive data sets.
- Support for Automation and High-Performance Computing.
- Robust Organization and Metadata support.
- 24/7 Support from the vendor.
WEKA: High-Performance Cloud Processing for Life Science Workloads
The core of a significant life science project is the technical system that supports it. This includes harnessing multiple data sources, processing them with high-performance computing, and pushing them through robust analytics and machine learning systems.
WEKA is a purpose-built platform to support these academic and research workloads in the life sciences. From genomic sequencing to pharmaceutical research and software development, WEKA can support all of these projects with its features and hardware:
- Streamlined and fast cloud file systems to combine multiple sources into a single high-performance computing system
- Industry-best GPUDirect performance (113 Gbps for a single DGX-2 and 162 Gbps for a single DGX A100)
- In-flight and at-rest encryption for governance, risk, and compliance requirements
- Agile access and management for edge, core, and cloud development
- Scalability up to exabytes of storage across billions of files
Contact the WEKA support team to learn more about our HPC cloud systems for life science workloads.
Additional Resources
- HPC for Life Sciences
- AI-based Drug Discovery with Atomwise and Weka on AWS
- Accelerating Genomic Discovery with Cost-Effective, Scalable Storage
- Accelerating Discovery and Improving Patient Outcomes with Next-Generation Storage
- How to Analyze Genome Sequence Data on AWS with WekaFS and NVIDIA Clara Parabricks
- Top 5 Myths in HPC for Life Sciences