What Happens When AI Meets Cloud Infrastructure?
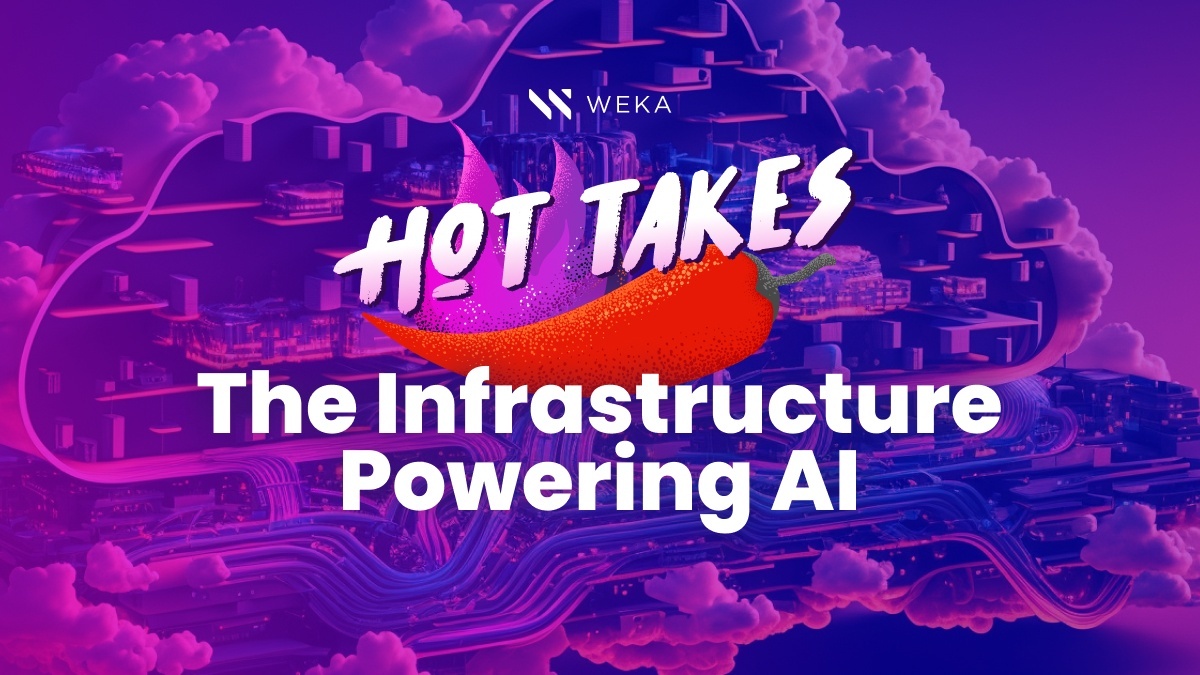
Making Sense of the Rapid Progress of AI Capabilities in the Cloud
“It is not the strongest of the species that survive, nor the most intelligent, but the one most responsive to change.” –Charles Darwin
The coming wave of AI applications will transform our world. This statement probably sounds a bit trite to anyone observing the recent explosion in AI-based applications and services. In just nine months since Chat GPT launched and inspired a new wave of innovation from start-ups and incumbents alike, there has been no shortage of examples of just how transformative AI will be. From education to content development to healthcare and public safety, it’s likely no part of our world will be left untouched by AI technologies.
The rapid innovation in AI applications will transform the current cloud computing landscape, just as it will every other industry, but it will do so in some surprising ways. Forward-thinking organizations are looking hard at how their current cloud strategy intersects with key investments they are making in AI.
It is now clear that the different cloud providers have dramatically different strategies for providing “full stack” AI tool sets for their customers. While it’s clear that the race to win AI is a marathon, we’re still only in the first 5k, and patterns are already beginning to emerge that will likely define how the rest of the race will play out. The cloud providers themselves are wary of this fact, just as in the marathon, you can’t win the race in the first 5k, but you can lose. There are three main criteria emerging that leading organizations are using to evaluate the AI race in the cloud: AI Infrastructure, AI developer tools, and AI-driven applications.
First up, let’s talk about AI Infrastructure:
GPUs reign supreme…for now
At the moment, the infrastructure game is all about compute, specifically, affordable access to the fastest graphical processing units (GPUs) that drive model training. At the moment, this puts NVIDIA in the driver’s seat with both the A100s most commonly available, and newer H100’s released from NVIDIA, now available on AWS and Google, and now in preview from Azure. Also in this space, NVIDIA’s intentions to partner with Oracle, Google, and Microsoft to bring the NVIDIA DGX Cloud offering to these cloud providers will give customers already familiar with the NVIDIA software stack and developer tools for AI a place to go in their preferred cloud, with AWS notably absent.
Given the pricing and cost concerns, it should be no surprise that GPU as a Service offerings from recent entrants like Coreweave, ATNorth, Lambda Labs, Foundry Technologies, and Applied Digital have rapidly captured attention for their potential to offer lower-cost access to GPUs. The interesting consideration here is the focus these providers have on offering bare metal access to GPU servers optimized for model training could be highly disruptive to the incumbent cloud providers.
Organizations are likely to start looking for alternatives to NVIDIA’s clear domination of the GPU space today. Intel Gaudi 2 and AMD MI3000 both offer the potential to be that alternative. Additionally, cloud providers are putting more focus on their custom silicon offerings with Google Cloud TPU v5e joining AWS Tranium and AWS Inferentia. In this area, the pressure on Microsoft to release details on Project Athena will only rise.
Beyond compute, high-performance data management is likely to emerge as the next frontier in the race to train larger models – with ever-increasing data sets and parameters – faster than ever. Up to 70% of an epoch happens before the GPU. Model training today involves lots of time spent copying data between systems for various stages of the data pipeline – NAS for persistent storage, local file systems or parallel file systems for fast storage, and object storage for archival data. As a result, it’s common to see GPU utilization between 30% to 50%. To date, most cloud providers have been quiet with respect to innovation to unlock the power of their GPUs by driving utilization up and dramatically reducing training times.
As the growth of enterprise AI shows no signs of slowing down, the race is on to boost AI capabilities in the cloud.