Unleashing the Power of AI: The Evolution of AI Native Data Infrastructure
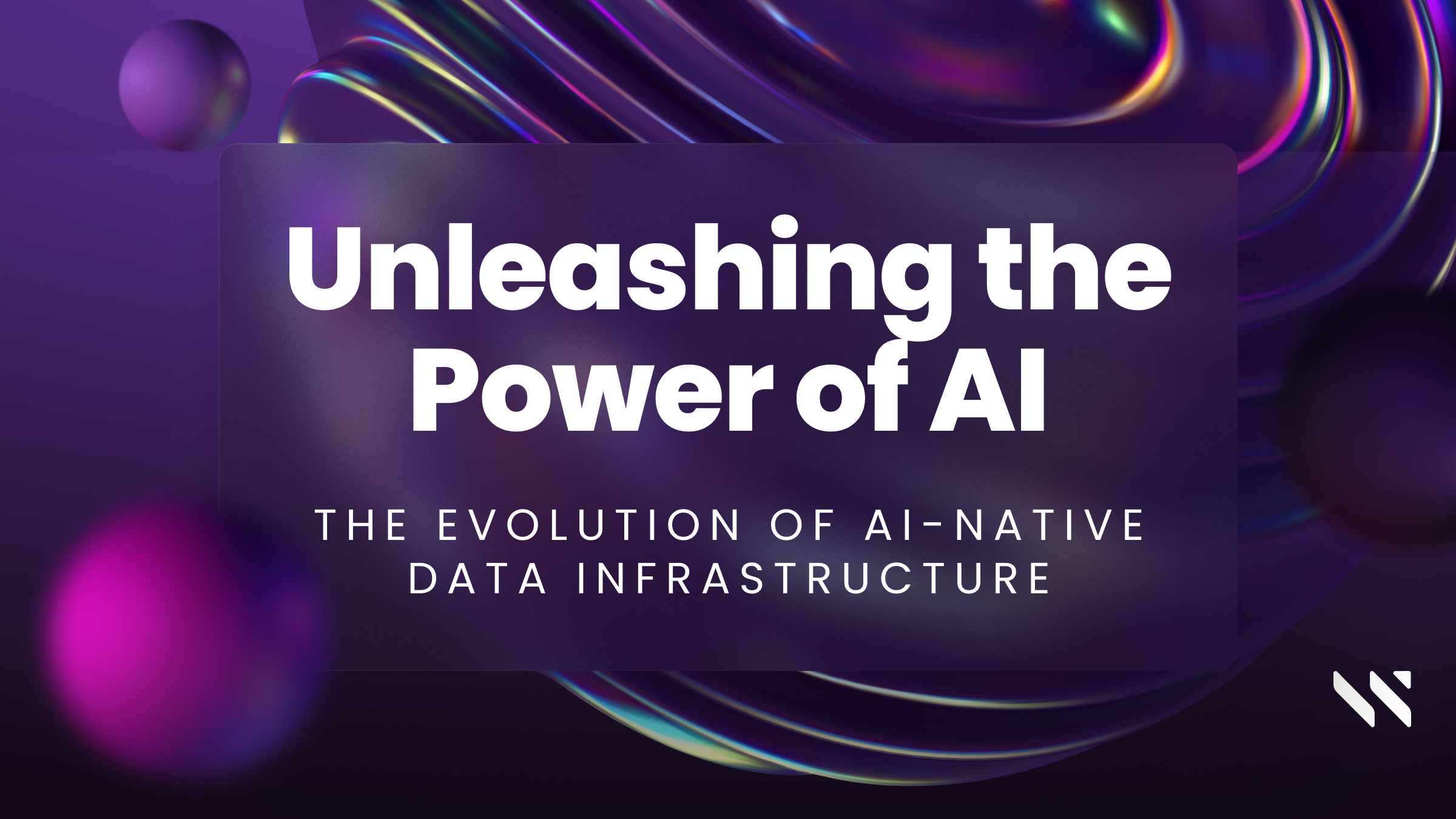
In today’s data-driven world, artificial intelligence (AI) has emerged as a transformative force across industries, revolutionizing how businesses operate, innovate, and deliver value. However, to realize AI’s full potential, a robust, modern data stack, specifically tailored to meet the unique IO patterns and performance demands of AI workloads is needed. These workloads present unique challenges from the scale and speed of data they use. Traditional storage systems are ill-equipped to meet the demands of modern AI workloads for several reasons.
Firstly, the scale of data processed by AI has reached unprecedented levels, with models handling trillions of parameters and datasets at a petabyte scale. Storage systems, designed for conventional data processing tasks, struggle to efficiently manage and access such vast volumes of data.
Secondly, the speed at which data needs to be processed has significantly increased with the advancement of AI algorithms and infrastructures. Despite orders of magnitude improvements in network speeds and GPU performance, legacy storage systems haven’t kept up and lack the necessary throughput and latency characteristics to support the rapid data access requirements of AI applications.
Moreover, the transition from training to inference exacerbates these challenges. In inference scenarios, where AI models make real-time predictions and decisions, the need for quick access to data becomes even more critical. Traditional storage systems may not be optimized to handle the instantaneous data retrieval demands of inference workloads, leading to performance bottlenecks and delays in decision-making processes.
Additionally, the evolving nature of AI techniques, such as expanding context windows and adopting advanced models like retrieval augmented generation (RAG), further strains traditional storage systems. These techniques require flexible and scalable storage solutions that can adapt to the changing requirements of AI workflows, which traditional storage architectures may struggle to provide.
The limitations of traditional storage systems in meeting the scale, speed, and agility requirements of modern AI workloads highlight the need for specialized AI native data infrastructure tailored to the unique demands of AI applications.
What is AI Native?
What does it really mean to be AI-Native? The term “AI native” has emerged in response to the growing recognition that traditional approaches to technology infrastructure are inadequate for supporting the demands of artificial intelligence (AI) systems. The concept of being “native” to AI implies that a technology or infrastructure is specifically designed and tailored to meet the needs of AI applications. This includes considerations such as scalability, flexibility, efficiency, and compatibility with the algorithms and processes commonly used in AI workflows.
When applied to data infrastructure, being AI native signifies more than just being able to run AI workloads, it denotes a fundamental reorientation of how data is collected, stored, and processed so that the wide variety of AI workloads can run efficiently at scale at a reasonable cost.
Understanding AI-Native Data Infrastructure
AI native data infrastructure refers to systems that can explicitly support the broad requirements of all types of AI applications. AI is a great umbrella term, but the various types of workloads (Natural Language Processing, Computer Vision, and other types of AI model development) can have very different demands for data. Unlike legacy data infrastructures, which may struggle to cope with the complexities of AI workloads, AI native infrastructure is purpose-built to handle large volumes of data, diverse data types, variable IO profiles, and high computational demands.
To be AI native, data infrastructure needs to deliver 5 key capabilities:
Scalability: AI native data infrastructure is designed to scale horizontally, effortlessly accommodating the ever-growing volumes of data generated by AI applications. Scaling is not just for capacity either. Scaling massive amounts of files, metadata, and clients in a linear fashion are other dimensions that need to be addressed.
Flexibility: Recognizing the diverse nature of AI data, AI native data infrastructure offers flexibility in handling the varying IO needs and the differing protocols required for data ingestion, storage, preprocessing, transformation, and training, which is crucial for AI workflows.
Performance: With optimized computational resources, AI native data infrastructure ensures high performance, which is critical for training and inference tasks in AI models. It also delivers low latency to enable AI applications to deliver timely insights and responses, which is essential for applications like predictive analytics and anomaly detection.
Cost- and energy-effectiveness: AI native data infrastructure offers cost-effective solutions, allowing organizations to manage AI workloads efficiently without incurring exorbitant infrastructure costs. It also helps to lower the energy and cooling costs associated with AI data pipelines.
Empowering AI Innovation: By providing a solid foundation for AI development and deployment, AI native data infrastructure empowers organizations to unleash AI’s full potential across various domains. Whether it’s enhancing customer experiences, optimizing operations, or driving strategic decision-making, AI native infrastructure is the backbone of AI-driven innovation.
WEKA, the AI Native Data Platform
WEKA’s clean sheet design for an AI data platform more than meets the criteria to be AI native. In 2013, we set out with a blank sheet of paper and a vision to create a product that would eradicate the compromises of the past AND power the possibilities of the future. The WEKA® Data Platform is purpose-built for large-scale AI whether on-prem or in multi-cloud environments. Its advanced architecture delivers radical performance, leading-edge ease of use, simple scaling, and seamless data sharing, so you can take full advantage of your enterprise AI workloads in virtually any location.
WEKA’s first customer deployed – and continues to use – WEKA for large-scale AI training. Since then we have continued to help many customers get their “AI wins.” More recently with the adoption of Generative AI, we have seen customers – including but not limited to Innoviz, ElevenLabs, Upstage, Stability AI, Midjourney, Samsung Labs, Cerence, and Adept AI – actively using WEKA to develop their various LLMs, latent diffusion, and other GenAI models. We also have a number of customers who host generative and predictive AI model development for other clients; this includes GPU cloud providers Applied Digital, IREN (formerly Iris Energy), NexGen Cloud, Sustainable Metal Cloud, and Yotta Data Systems.
As AI continues to evolve and permeate every aspect of our lives, the importance of AI native data infrastructure cannot be overstated. By embracing AI native infrastructure, organizations can harness the power of AI to drive growth, competitiveness, and value creation in today’s data-centric world. With built-in scalability, flexibility, high performance, sustainability, and cost-effectiveness at its core, our cloud, and AI-native WEKA® Data Platform is helping to modernize the enterprise data stack to fuel the next wave of intelligent insights and AI innovations.