WEKA Sets New Records with Unofficial MLPerf Storage v0.5 Benchmark
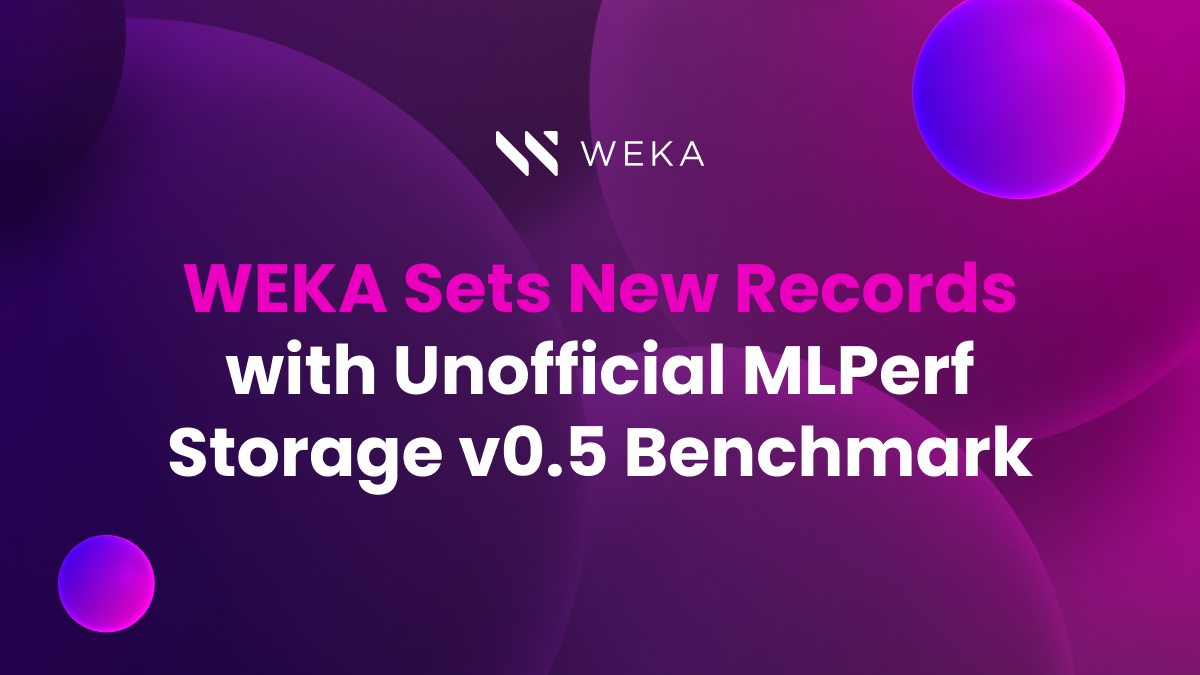
MLPerf Storage is a relatively new addition to the MLPerf suite of benchmarks, designed to evaluate and measure the performance of storage systems in machine learning (ML) and artificial intelligence (AI) workloads. Version 0.5 was introduced last year as part of the MLCommons efforts to provide standardized benchmarks for evaluating the performance of ML and AI systems across different hardware and software platforms. And while version 1.0 will debut shortly and supersede it, we wanted to unofficially run the MLPerf Storage v0.5 benchmark in a cloud environment to preview both the power of the WEKA® Data Platform for AI workloads and WEKA’s alignment with the strategic cloud-first approach of AI innovators who leverage cloud elasticity and scale for extensive model training.
Benchmark Significance
The MLPerf Storage Benchmark Suite, maintained and developed by MLCommons, is part of a collection designed to foster the next generation of AI model development. The suite assesses storage performance by simulating NVIDIA® V100 accelerators, providing a common platform to compare different storage solutions. Version 0.5 of the suite introduced benchmarks for two models: Unet-3D, used for image segmentation, and BERT, a natural language processing model. These benchmarks are designed to challenge and measure the storage capabilities required for state-of-the-art AI applications.
WEKA’s Cloud Strategy
Following a compelling single-client test of MLPerf Storage last November using a small cluster, WEKA decided to undertake the MLPerf Storage v0.5 benchmark in the cloud. This decision was driven by a desire to mirror the real-world production environment scenarios of WEKA AI customers like Stability AI, NexGen Cloud, and Applied Digital.
These AI trailblazers utilize cloud environments to capitalize on available flexible resources and extensive networking capabilities for expansive AI model training. WEKA’s MLPerf cloud benchmark, therefore, not only serves as an indicator of performance but also as a testament to the cloud adaptability of our high-performance WEKA Data Platform.
Unveiling Performance Advantages in Cloud-Based AI Benchmarking
WEKA’s cloud-based MLPerf Storage benchmark leveraged 64 clients using c5n.18xl shared instances and i3en.24xl backend instances on AWS. This setup replicates the distributed client deployment scenarios typical of supporting cloud-based AI workloads, only without GPUs, as the MLPerf Storage benchmark does not require instances with GPUs. This cloud-native approach underscores WEKA’s dedication to performance and scalability, standing in stark contrast to the current MLPerf Storage v0.5 benchmark record set by another storage company using high-speed InfiniBand in an isolated lab environment.
Exceptional Scalability and Throughput in the Cloud
WEKA’s Data Platform exhibited remarkable scalability and throughput within the cloud environment. For the Unet3D model, which is a 3D medical imaging model that reads large image files into accelerator memory, WEKA achieved a new record of 1280 simulated V100 GPUs, an impressive eight-fold increase over the current record.
During the testing, the clients easily achieved 10GB/s of throughput with the WEKA Data Platform sustaining over 600GB/s, 5M IOPS, at 1ms latency!
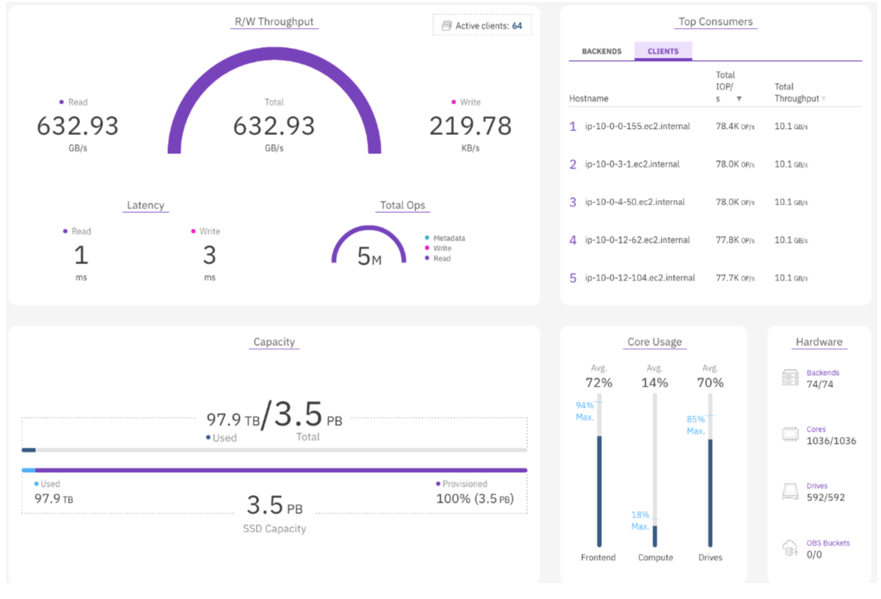
WEKA’s performance dominance was even more pronounced with the BERT model. The system successfully set a new record of 2560 simulated GPUs, a sixteen-fold increase.
Unet-3D WEKA in cloud vs. Competitor on-premises
Competitor’s on-premises: | WEKA in the cloud: | Results: | |
Accelerators | 160 | *1280 | WEKA 8x more |
Samples/sec | 441 | *3413 | WEKA 7.7x more |
* Result not verified by MLCommons Association.
BERT WEKA in the cloud vs. Competitor on-premises
Competitor’s on-premises | WEKA in the cloud | Results: | |
Accelerators | 160 | * 2560 | WEKA 16x more |
Samples/sec | 7885 | * 115932 | WEKA 14.7x more |
* Result not verified by MLCommons Association.
The Optimal Choice for AI Workloads in the Cloud
The WEKA Data Platform’s remarkable cloud performance signifies more than just impressive figures. It translates to a superior ability to support AI model training at a scale that reflects real-world customer practices. WEKA’s integration with cloud operations showcases its preparedness to lead in the upcoming surge of AI innovations and the demands for high-performance storage.
Transparency and Future Considerations
It’s important to acknowledge that the results presented here are outside the MLCommon submission window for MLPerf Storage v0.5 results and are therefore unvalidated. However, they are indicative of WEKA’s immense performance potential in cloud environments.
While MLPerf Storage v1.0 is on the horizon, these results remain faithful to the v0.5 benchmark, capturing the essence of a distributed AI training landscape within the cloud. WEKA will submit MLPerf Storage v1.0 results when it becomes available in May 2024.
Leading the Charge in Cloud-based Storage Performance for AI Workloads
WEKA’s record-setting performance with MLPerf Storage v0.5 and other industry benchmarks and the growing number of WEKA customers running AI workloads in the cloud signifies a pivotal shift for AI and ML workloads deployed in the cloud.
With the growing complexity of AI models necessitating unprecedented levels of distribution and demanding heightened storage capabilities, WEKA’s Data Platform emerges as a critical solution. Its role in providing necessary performance is more significant than at any previous point. Demonstrating exceptional scalability and efficiency, WEKA’s Data Platform sets a standard for managing AI workloads.
The MLPerf name and logo are registered and unregistered trademarks of MLCommons Association in the United States and other countries. All rights reserved. Unauthorized use is strictly prohibited. See www.mlcommons.org for more information.