Why AI Needs a Data Platform
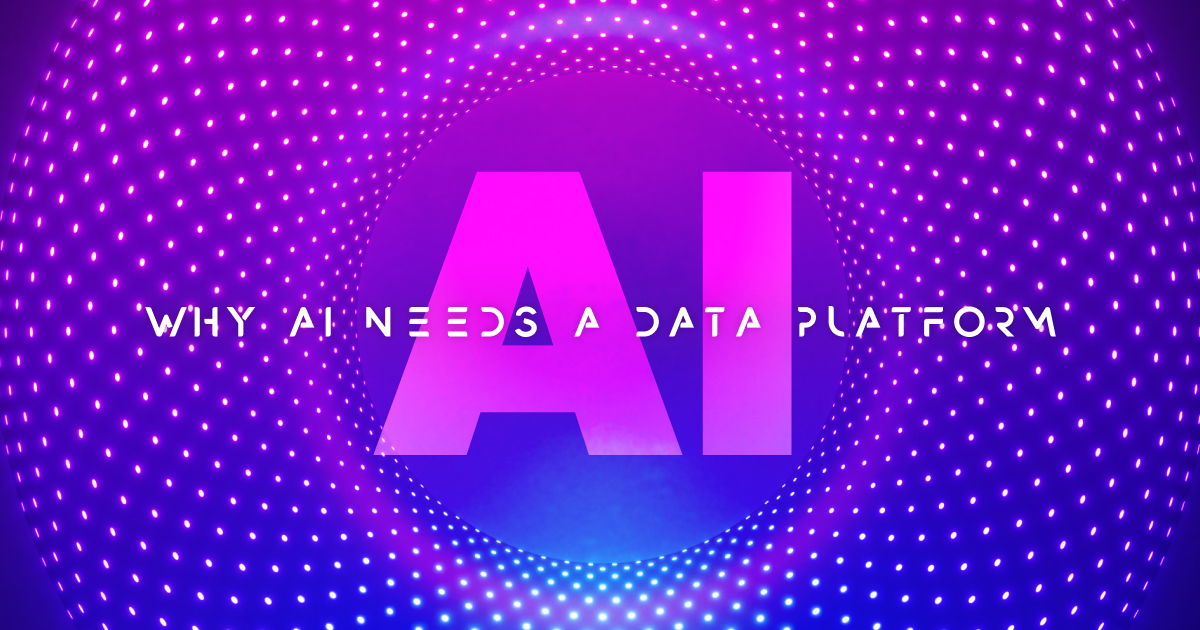
The term “digital transformation,” although widely used, has so far proven to be something of a misnomer. Although businesses have quite successfully digitalized many formerly analog processes and achieved valuable efficiencies and process improvements as a result, most have not been fundamentally transformed by these changes. Their tools may look new, but effectively they’re still running business as usual with a digital veneer atop of legacy processes.
This is about to change. With the confluence of massive amounts of data and ready access to the higher orders of compute power offered by modern GPUs, enterprise technology is now reaching a critical inflection point thanks to artificial intelligence (AI), which has the potential to be truly transformative at enterprise scale, be it on premises or in the cloud. AI has the ability to see patterns, create detailed models of behavior, and make predictions that will refactor business processes in a major way.
What does transformation look like?
Consider the automobile. For the better part of a century, cars were fully analog. Then, over decades, digital services began to appear: electronic sensors fed information to digital gauges; cameras began to read lane markings, automatically nudging the steering wheel to keep the car in its lane; radar enabled adaptive cruise control to maintain follow distance on the highway automatically. But while discrete systems were becoming digital, the vehicles themselves remained largely unchanged until recently, when a new layer controlled by AI made self-driving cars possible. Suddenly, the very nature of the automobile has been altered, removing the driver from the equation and freeing people to do other things—fundamentally transforming what a car has been until now.
Many organizations are now exploring and investing in AI-based initiatives to automate repetitive processes, improve customer experience, reduce costs, and provide support for their workers. We are also seeing AI applications that address specific business intelligence (BI) and high performance computing (HPC) challenges. But even within these narrow confines, industry analysts report that only 50% of AI models ever make it to production1.
There are a variety of factors contributing to this of course, but one prominent barrier is that most organizations’ data infrastructure is simply not equipped to support the extreme data processing and performance demands of AI workloads.
Is your AI initiative data starved?
For AI to power the next evolutionary leap in business transformation or research innovation, it needs to be able to do more. AI can support enterprise scale requirements – if the data is there. But without a modern data infrastructure that can rapidly process massive volumes of data, it can’t deliver on its full promise and potential.
Transformative AI needs data infrastructure that can organize and feed it data without all the hand-crafted artisanal work generally required with AI projects today, which are hampered by slow data pipelines. Recent has research found that AI GPU accelerators can spend up to 70% of their time idle2, waiting for data. It requires a new kind of data infrastructure that is purpose-built to fuel massive quantities of data at low latencies.
Speed is critically important to the AI-fueled enterprise. Building, training, and improving AI models is an iterative process. Efficiently feeding the right data to the system is the difference between a project requiring months versus mere days.
Implementing Transformative AI
Organizations are starting to understand that integration and ecosystem complexity are big challenges. Legacy infrastructure cannot integrate effectively with AI to deliver the next generation, transformative results they need, so they are investing in faster networks to deliver data. GPU accelerators are recognized as being better suited to running AI models than CPUs. The next critical step will be to move from traditional storage to a data platform suited to AI.
WEKA provides that data platform. Each one of the steps of a data pipeline usually has a completely different profile for what the data looks like. This can cause issues with traditional storage, which is tuned to address one data type or throughput performance profile. Some steps need low latency, random small IO, others need massive streaming throughput; still others need a concurrent mix of the two because of sub-steps within the process. In most environments, multiple pipelines will run concurrently, but at different stages, amplifying the need to handle different IO profiles simultaneously
The WEKA Data Platform for AI performs across all dimensions without the need for tuning or re-configuration. Customers can run any part of their data pipeline on a single system – whether it requires massive IOPS with small reads and writes or massive throughput and 10’s to 100’s of GB/sec.
But the WEKA Data Platform delivers more than just speed – it also builds efficiency into your AI environment, providing a single platform that simplifies and accelerates the entire data pipeline to meet the most demanding performance requirements, regardless of the workload.
Strategic Infrastructure
AI is now powering massive enterprise-scale deployments that are supporting previously unimaginable scientific and technological breakthroughs. As companies of all sizes across every industry are now looking to use AI to harness their data and fundamentally transform their businesses, having the right data infrastructure in place has never been more important. Accelerated compute, fast networking, and high speed data platforms like the WEKA Data Platform for AI, are rapidly becoming a strategic imperative to run AI at enterprise scale.
1Gartner, Inc., A CTO’s Guide to Top Artificial Intelligence Engineering Practices, Arun Chandrasekaran, Farhan Choudhary, Erick Brethenoux, 29 October 2021
2Characterization and Prediction of Deep Learning Workloads in Large-Scale GPU Datacenters