Surveying the Secrets of Successful AI Adoption
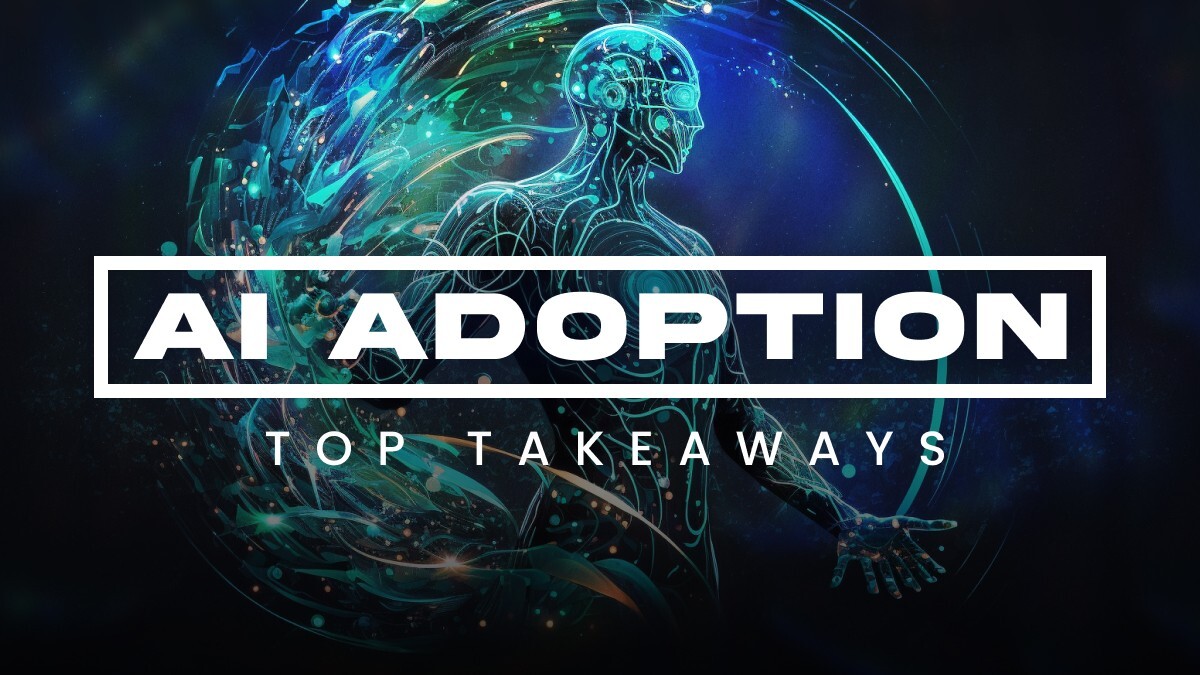
Most of us hate surveys. They are time-consuming, interrupt our days, and are often used to ultimately sell us something. Conversely, a well-conducted survey provides a structured way to collect information across a large group of people and can offer insights into consumer preferences, political opinions, market trends, and more. In the technology industry, surveys play an important role in gathering insight about the use of emerging technologies that can help us spot emerging trends that will make a significant impact on global markets, industries, or society.
AI is experiencing exponential growth and remarkable diversification across various sectors. From healthcare and finance to manufacturing and entertainment, AI technologies are rapidly evolving, transforming industries in profound ways. Machine learning, natural language processing, computer vision, and robotics are becoming integral parts of business operations, enhancing decision-making, automating processes, and driving efficiency. As AI algorithms become more advanced and accessible, organizations are harnessing their power to analyze enormous datasets, personalize user experiences, optimize supply chains, and even make strides in scientific research.
Despite this Cambrian explosion of AI today, many organizations are still in the early stages of adopting it. To help them understand what has helped – and hindered – the success of early AI pioneers, WEKA recently partnered with S&P Global Market Intelligence to conduct one of the largest global studies of AI technology decision-makers and practitioners to date.
There are some incredible takeaways and deep lessons in the data gathered on how rapidly AI/ML is transforming businesses across industries and its potential future uses, but also the challenges organizations face as they look to use it at enterprise scale. We encourage you to read our summary report for all of these learnings. In this blog, however, I want to dive into two key findings that I found most interesting – the top technical inhibitors to AI/ML adoption and how to leverage a hybrid approach to AI/ML deployment – and lessons organizations can learn from them.
AI Presents Data Management Challenges
It was no surprise that many participants reported that their organization’s IT infrastructure and data architectures are unfit for the new AI workloads. Their continually growing demands are placing significant strain on legacy enterprise IT systems and data architectures. While IT media stories have focused mainly on the costs and accessibility of GPUs of late, the survey revealed a broader range of obstacles, most related to effective data management. Data is the lifeblood of AI, yet without proper oversight, it can swiftly become a hindrance. Grappling with the immense data volumes needed for AI model training poses a major challenge. As businesses position themselves to comprehensively address data management, they encounter further complexities arising from the increasingly intricate landscape of deployment sites, use scenarios, and stakeholders.
While customers have been saying for years that their legacy IT infrastructure and data architectures are unfit for the AI revolution and that they need new approaches, it was surprising to see how high they ranked data management challenges -–#1. Data management outweighed other important and more typically cited infrastructure challenges, like data security and compute performance.
The respondents called out a variety of reasons why, including challenges with data sourcing, preparation, standardization, insufficient IT infrastructure to handle unwieldy data volumes, the unique requirements for structured data, unstructured text and streaming/real-time data, and the challenge of data silos.
The lesson here is that companies looking to deploy or expand their AI initiatives should look at the surprising emphasis the respondents placed on data management challenges and assess their infrastructure for its AI workload readiness.
AI Has Gone Hybrid
The second thing that struck me was the vast number of deployment locations and that there was no clear “right” choice. While often discussed simplistically – “should AI be deployed on-premises in the cloud?” The choice between deploying AI on-premises or in the cloud varies based on factors such as organizational requirements, scalability needs, data sensitivity, and resource availability. On-premises deployments offer greater control over data and security, while the cloud provides greater scalability, flexibility, and reduced infrastructure overhead. Both options have their merits, and the decision to leverage one or the other often depends on specific use cases and business priorities.
But for the respondents of this study, deployment location was not a simple binary question. Their organizations are using multiple locations and clouds for AI workloads. The data shows that the public cloud is often, but not exclusively, a go-to starting point and the top choice for training AI/ML models for data-heavy and complex AI applications. But as these models go through different phases, they need to be processed in different places. That’s where a hybrid approach takes over. The more mature the AI deployment, the more likely an organization is to use multiple locations to develop, train, and deploy AI/ML models. AI pioneers, with one of more production workloads, reported using 3.2 deployment locations on average.
Here, the lesson is that companies should be incorporating hybrid cloud into the their AI deployment planning from the outset given they will likely evolve to using multiple locations in the future, they should choose solutions that can facilitate frictionless data sharing across cloud and on-premises environments when the need arises.
Mine the Data Yourself
These are just two of the seven major takeaways from this expansive study from S&P Global. It has numerous other insights on how to organizations can successfully scale and grow their AI deployments, on what defines an AI pioneer, and lessons on how companies can use AI more sustainably to support corporate sustainability goals (CSGs).
Download and read the full report today to guide and accelerate your own AI initiatives.